The pharmaceutical industry has always been at the forefront of scientific innovation, constantly seeking new ways to develop and bring to market life-saving and life-improving drugs. In recent years, the rise of artificial intelligence (AI) has brought about a profound transformation in the drug discovery and development process. This article explores the pivotal role of AI in this critical field, delving into the latest developments, applications, and future prospects.
Introduction
The drug discovery and development process is a complex, time-consuming, and resource-intensive endeavor. Traditional methods have often been hindered by the sheer volume of data, the need for meticulous experimentation, and the inherent challenges of identifying potential drug candidates. However, the advent of AI has ushered in a new era, promising to streamline and accelerate this process, ultimately leading to more effective and efficient drug discoveries.
Overview of Drug Discovery and Development
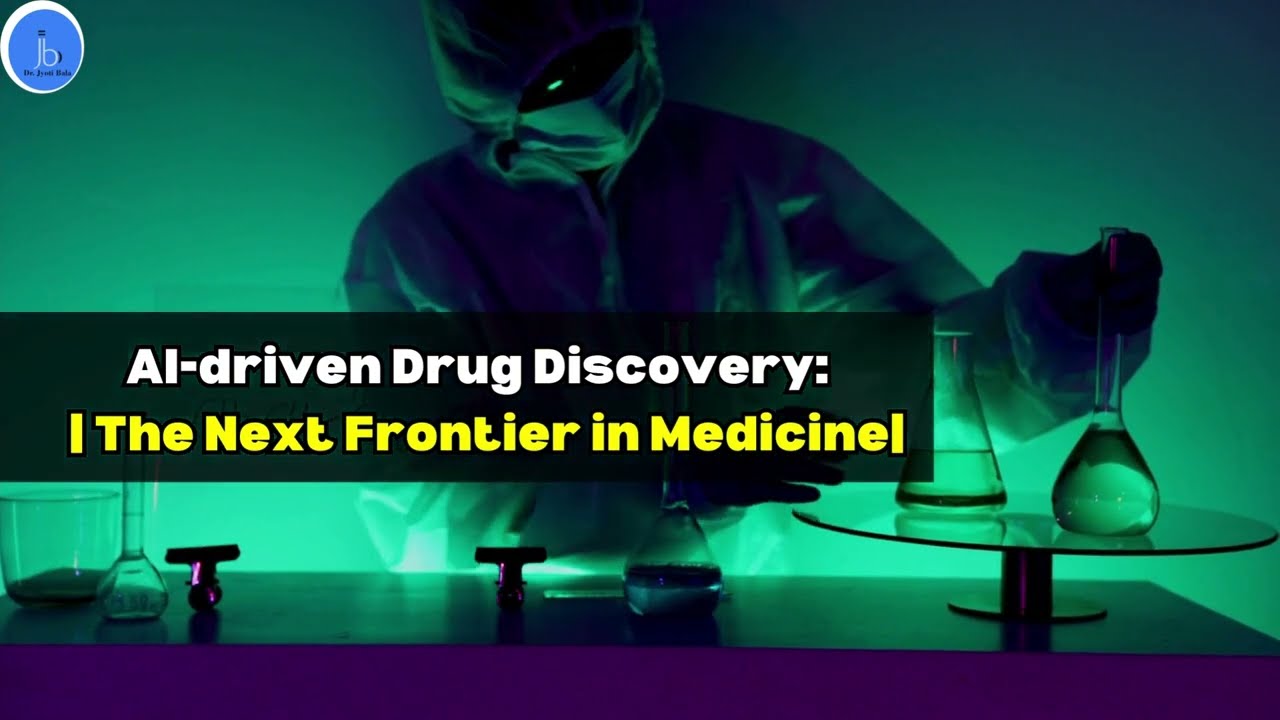
The drug discovery and development process is a multi-step journey that involves the following key stages:
Target Identification and Validation
The process begins with the identification of a specific biological target, such as a protein or enzyme, that is believed to play a crucial role in a particular disease or condition. Researchers then validate the target’s relevance and potential for therapeutic intervention.
Lead Compound Identification
Once a target has been identified, researchers must search for and evaluate potential drug candidates, known as “lead compounds,” that can interact with the target and potentially produce the desired therapeutic effect.
Preclinical Testing
The lead compounds undergo extensive preclinical testing, including in vitro (laboratory) and in vivo (animal) studies, to assess their safety, efficacy, and potential for further development.
Clinical Trials
Compounds that pass the preclinical stage are then tested in a series of clinical trials involving human participants. These trials are designed to evaluate the drug’s safety, dosage, and effectiveness in treating the target condition.
Regulatory Approval
If a drug candidate successfully navigates the clinical trial process, it is then submitted to regulatory authorities, such as the U.S. Food and Drug Administration (FDA) or the European Medicines Agency (EMA), for review and approval.
Post-Marketing Surveillance
Even after a drug is approved and made available to the public, ongoing monitoring and data collection are crucial to ensure its continued safety and effectiveness.
Importance of AI in the Pharmaceutical Industry

The pharmaceutical industry has long been at the forefront of technological advancements, and the emergence of AI has proven to be a game-changer. AI technologies offer the potential to revolutionize the drug discovery and development process in several key ways:
Enhanced Data Analysis
AI-powered algorithms can analyze vast amounts of complex data, including chemical structures, biological information, and clinical trial results, far more efficiently than human researchers. This allows for the identification of patterns, trends, and insights that may have been previously overlooked.
Improved Target Identification
AI can assist in the identification and validation of novel drug targets by analyzing massive datasets, including genomic information, disease pathways, and biological networks.
Accelerated Lead Compound Identification
AI-driven computational models can rapidly screen and evaluate millions of potential drug candidates, significantly reducing the time and resources required to identify promising lead compounds.
Enhanced Preclinical Testing
AI can be leveraged to optimize and streamline preclinical testing, such as predicting drug toxicity, simulating in vivo experiments, and identifying the most promising candidates for further development.
Optimized Clinical Trials
AI can help design and manage clinical trials more effectively, from patient recruitment and data analysis to the identification of subgroups most likely to benefit from a particular drug.
Personalized Medicine
By integrating AI with genomic and clinical data, the pharmaceutical industry can develop more personalized and targeted treatments, improving patient outcomes and reducing the risk of adverse reactions.
AI Technologies Used in Drug Discovery
The pharmaceutical industry has embraced a wide range of AI technologies to enhance the drug discovery and development process. Some of the key AI techniques and their applications include:
Machine Learning
Machine learning algorithms can analyze large datasets, identify patterns, and make predictions that can inform the drug discovery process. This includes techniques such as supervised learning, unsupervised learning, and reinforcement learning.
Deep Learning
Deep learning, a specialized form of machine learning, has shown exceptional promise in areas such as drug target identification, molecular structure prediction, and drug repurposing.
Natural Language Processing (NLP)
NLP techniques can extract valuable insights from the vast troves of scientific literature, patents, and clinical trial data, helping researchers stay abreast of the latest developments in the field.
Generative Models
Generative AI models, such as generative adversarial networks (GANs) and variational autoencoders (VAEs), can create novel molecular structures and designs, expanding the pool of potential drug candidates.
Quantum Computing
Emerging quantum computing technologies have the potential to simulate the complex interactions between molecules and proteins, accelerating the drug discovery process.
Robotics and Automation
AI-powered robotic systems can automate various laboratory tasks, from high-throughput screening to experimental design, improving efficiency and reducing human error.
Applications of AI in Drug Development
AI’s impact on the drug development process extends beyond the discovery phase, with numerous applications in the various stages of the pipeline:
Target Identification and Validation
AI algorithms can analyze vast amounts of genomic, proteomic, and disease-related data to identify and validate novel drug targets with greater accuracy and speed.
Lead Compound Screening and Optimization
AI-driven computational models can rapidly screen chemical libraries, identify promising lead compounds, and optimize their molecular structures for improved potency and safety.
Preclinical Testing and Toxicology
AI can be used to predict drug toxicity, simulate in vivo experiments, and identify the most promising candidates for further development, reducing the need for costly and time-consuming animal studies.
Clinical Trial Design and Patient Recruitment
AI algorithms can help design more efficient clinical trials, optimize patient recruitment, and identify subgroups most likely to benefit from a particular drug.
Drug Repurposing
AI can be leveraged to identify new therapeutic uses for existing drugs, a process known as drug repurposing, which can significantly accelerate the development of new treatments.
Personalized Medicine
By integrating AI with genomic, clinical, and real-world data, the pharmaceutical industry can develop more personalized and targeted treatments, improving patient outcomes and reducing the risk of adverse reactions.
Case Studies of Successful AI-Driven Drug Discoveries
The pharmaceutical industry has already witnessed several notable success stories in the application of AI for drug discovery and development. Here are a few examples:
Case Study 1: Designing a Novel Antibiotic Using AI
In 2020, researchers from the Massachusetts Institute of Technology (MIT) and the University of Cambridge used AI to design a novel antibiotic compound, named “halicin,” that was effective against a wide range of drug-resistant bacteria, including Escherichia coli and Acinetobacter baumannii. The AI-driven design process was significantly faster and more efficient than traditional methods.
Case Study 2: Repurposing Existing Drugs Using AI
Researchers at the University of Cambridge and the Drugs for Neglected Diseases initiative (DNDi) used AI to identify existing drugs that could be repurposed to treat COVID-19. The team’s AI models analyzed millions of potential drug candidates and identified several existing drugs, including the antidepressant fluvoxamine, that showed promising results in clinical trials.
Case Study 3: Predicting Drug-Target Interactions Using AI
Researchers at the University of Toronto and the Hospital for Sick Children (SickKids) developed an AI-powered platform called “DeepDTA” that can accurately predict drug-target interactions. This technology has the potential to significantly accelerate the identification of novel drug candidates and the optimization of existing drugs.
Challenges and Limitations of AI in Drug Discovery
While the pharmaceutical industry has embraced the transformative potential of AI, there are still several challenges and limitations that need to be addressed:
Data Availability and Quality
The effectiveness of AI-driven drug discovery relies heavily on the availability and quality of the underlying data. Insufficient or low-quality data can lead to inaccurate predictions and suboptimal results.
Interpretability and Transparency
Many AI models, particularly those based on deep learning, are often referred to as “black boxes,” making it difficult to understand the reasoning behind their predictions. This lack of interpretability can be a barrier to the adoption of AI in the highly regulated pharmaceutical industry.
Validation and Regulatory Approval
Demonstrating the reliability and robustness of AI-driven drug discoveries is crucial for regulatory approval. Rigorous validation and testing procedures are necessary to ensure the safety and efficacy of AI-developed drugs.
Ethical Considerations
The use of AI in drug discovery raises ethical concerns, such as the potential for algorithmic bias, the protection of patient privacy, and the equitable distribution of AI-derived drugs.
Technical Challenges
Developing and deploying AI systems in the pharmaceutical industry comes with technical challenges, including the integration of AI with existing workflows, the management of large and complex datasets, and the need for specialized expertise.
Future Prospects of AI in Drug Discovery and Development
Despite the challenges, the future prospects of AI in the pharmaceutical industry are highly promising. As the technology continues to evolve and the industry gains more experience in its application, we can expect to see even greater advancements in the coming years:
Increased Collaboration and Data Sharing
Collaboration between pharmaceutical companies, academia, and technology firms will be crucial to addressing the data and expertise challenges. Increased data sharing and the development of shared AI platforms can drive further progress.
Advancements in AI Techniques
Ongoing developments in AI, such as the emergence of more interpretable and explainable models, the integration of reinforcement learning, and the application of quantum computing, will further enhance the capabilities of AI in drug discovery.
Improved Regulatory Frameworks
As the use of AI in the pharmaceutical industry becomes more widespread, regulatory bodies are likely to develop clear guidelines and frameworks to ensure the responsible and ethical deployment of these technologies.
Personalized and Precision Medicine
The integration of AI with genomics, real-world data, and advanced analytics will enable the pharmaceutical industry to develop increasingly personalized and targeted treatments, leading to improved patient outcomes.
Accelerated Drug Discovery Timelines
The continued adoption of AI in drug discovery and development is expected to significantly reduce the time and costs associated with bringing new drugs to market, ultimately benefiting patients and healthcare systems.
Conclusion
The role of AI in the pharmaceutical industry is undeniably transformative, with the potential to revolutionize the drug discovery and development process. By harnessing the power of advanced AI technologies, the industry can overcome longstanding challenges, accelerate the identification of promising drug candidates, and ultimately bring more effective and personalized treatments to patients in need.
As the pharmaceutical industry continues to embrace AI, the future of drug discovery and development looks increasingly promising. By overcoming the current challenges and leveraging the full potential of these cutting-edge technologies, the industry can pave the way for a new era of therapeutic breakthroughs, ultimately improving the lives of countless individuals worldwide.