In the face of growing environmental challenges, the use of artificial intelligence (AI) has emerged as a promising tool in the field of wildlife conservation. As biodiversity declines and the need for effective monitoring and protection of endangered species becomes increasingly urgent, AI offers innovative solutions that can revolutionize the way we approach conservation efforts.
Importance of AI in Wildlife Conservation
Enhancing Biodiversity Monitoring
Accurate and comprehensive biodiversity monitoring is crucial for understanding the health and status of wildlife populations. AI-powered technologies, such as machine learning and computer vision, can significantly improve the process of data collection and analysis.
- Automated Species Identification: AI-based algorithms can be trained to accurately identify different species from images or audio recordings, allowing for more efficient and scalable wildlife monitoring.
- Real-Time Monitoring: AI-powered systems can process data from various sensors, drones, and camera traps in real-time, enabling rapid detection of changes in wildlife populations and the environment.
- Predictive Analytics: AI algorithms can analyze historical and current data to predict future trends, potential threats, and optimal conservation strategies, helping to guide decision-making.
Enhancing Anti-Poaching Efforts
Poaching remains a significant threat to many endangered species. AI can play a vital role in enhancing anti-poaching efforts by improving surveillance, detection, and response capabilities.
- Automated Surveillance: AI-powered camera systems and drones can continuously monitor vast areas, detecting and tracking the movement of potential poachers.
- Predictive Modeling: AI algorithms can analyze patterns in poaching incidents and environmental data to predict high-risk areas, enabling more targeted and efficient deployment of anti-poaching patrols.
- Intelligent Decision Support: AI-powered systems can integrate various data sources, such as ranger reports, satellite imagery, and sensor data, to provide real-time recommendations for optimal patrol routes and resource allocation.
Improving Wildlife Habitat Management
Effective habitat management is essential for the long-term survival of wildlife species. AI can contribute to improved habitat monitoring, restoration, and conservation planning.
- Habitat Mapping and Monitoring: AI-powered remote sensing and satellite imagery analysis can help map and monitor the health and extent of wildlife habitats over time.
- Predictive Habitat Modeling: AI algorithms can use various environmental and ecological data to model and predict the suitability of habitats for specific species, guiding conservation efforts.
- Automated Habitat Restoration: AI can be used to optimize the planning and implementation of habitat restoration projects, ensuring efficient and effective outcomes.
Enhancing Community Engagement and Decision-making
Successful wildlife conservation efforts often require the involvement and cooperation of local communities. AI can support this by improving communication, engagement, and decision-making processes.
- Human-AI Collaboration: AI-powered tools can facilitate the collection and integration of local ecological knowledge, empowering communities to participate in conservation initiatives.
- Visualization and Simulation: AI-based visualizations and simulations can help stakeholders, policymakers, and the public better understand the impact of conservation actions and trade-offs.
- Personalized Outreach: AI can be used to develop targeted educational and awareness campaigns, tailoring the messaging and delivery to different community demographics.
Current AI Technologies Being Used
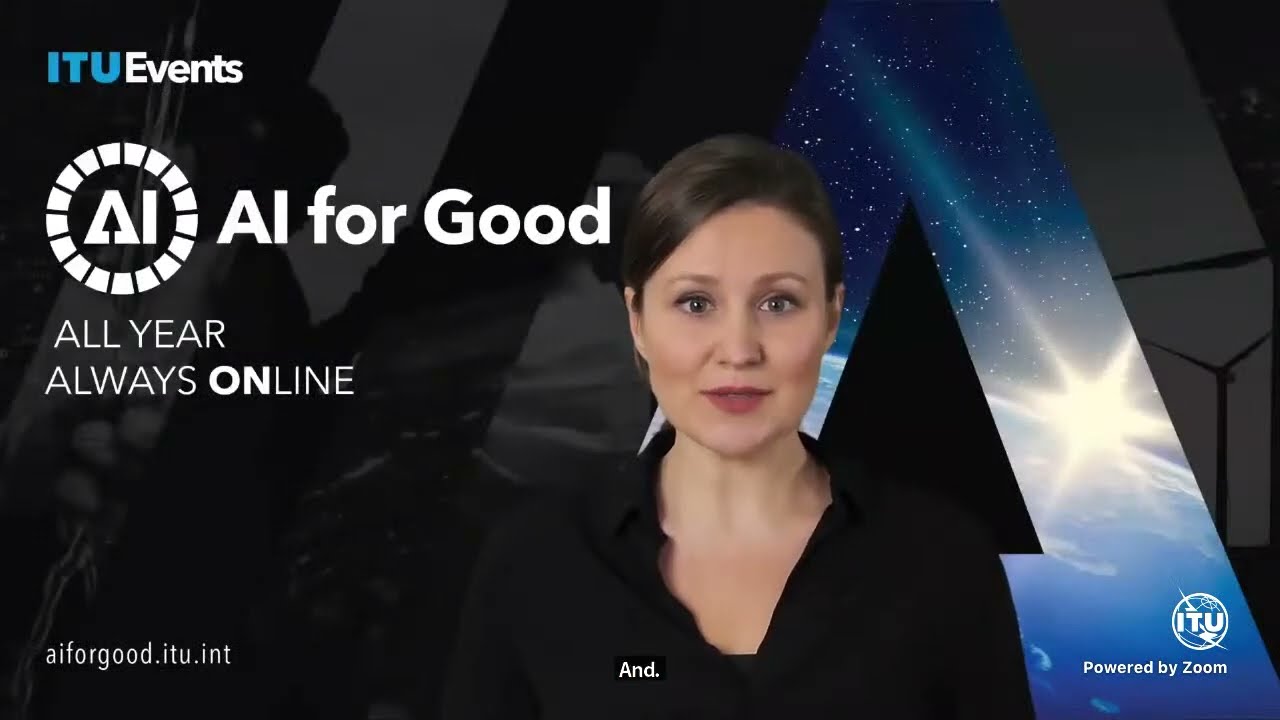
Computer Vision and Image Recognition
One of the most widely adopted AI technologies in wildlife conservation is computer vision and image recognition. These techniques allow for the automated identification and classification of species from images and videos, greatly enhancing the speed and accuracy of biodiversity monitoring.
- Camera Trap Monitoring: AI-powered algorithms can analyze images and videos captured by camera traps, detecting and identifying the presence of different species, as well as tracking their movements and behaviors.
- Aerial Imagery Analysis: AI can be used to process aerial and satellite imagery, detecting and mapping the distribution of wildlife, their habitats, and potential threats such as deforestation or illegal activities.
- Wildlife Tracking: AI-based computer vision algorithms can be trained to track individual animals, providing valuable insights into their movements, migration patterns, and interactions.
Audio Recognition and Bioacoustics
In addition to visual data, AI can also be applied to audio recordings to identify and monitor wildlife species based on their vocalizations.
- Automated Species Identification: AI-powered bioacoustic models can recognize and classify the unique calls and sounds of different animal species, enabling more comprehensive biodiversity monitoring.
- Acoustic Monitoring: AI-based systems can continuously record and analyze audio data from the environment, detecting changes in the acoustic landscape that may indicate the presence or absence of certain species.
- Soundscape Analysis: AI algorithms can be used to study the entire acoustic environment, providing insights into the overall health and diversity of the ecosystem.
Predictive Modeling and Decision Support
AI-powered predictive modeling and decision support systems are increasingly being used to inform and guide wildlife conservation efforts.
- Habitat Suitability Modeling: AI algorithms can integrate various environmental and ecological data to model and predict the suitability of habitats for specific species, helping to identify areas for conservation and restoration.
- Threat Identification and Prioritization: AI can analyze multiple data sources, such as satellite imagery, sensor data, and historical records, to identify and prioritize potential threats to wildlife, enabling more targeted and efficient conservation actions.
- Optimization and Resource Allocation: AI-based decision support systems can help optimize the allocation of limited resources, such as funding, personnel, and equipment, to maximize the impact of conservation initiatives.
Robotics and Autonomous Systems
Advancements in robotics and autonomous systems have also found applications in wildlife conservation, enabling new approaches to data collection, monitoring, and intervention.
- Unmanned Aerial Vehicles (UAVs): AI-powered drones and UAVs can be used for aerial surveys, anti-poaching patrols, and habitat mapping, providing a bird’s-eye view of the landscape.
- Autonomous Monitoring Stations: AI-enabled sensor networks and monitoring stations can be deployed in remote areas, continuously collecting and transmitting data on various environmental and wildlife parameters.
- Robotic Interventions: In some cases, AI-controlled robots or autonomous systems can be used for tasks like animal handling, sample collection, or habitat restoration, reducing the need for human intervention and minimizing disturbance to wildlife.
Case Studies of Successful AI Applications in Wildlife Conservation

Elephant Poaching Detection in Africa
In Kenya, a team of researchers and conservationists have developed an AI-powered system to detect and track elephant poaching activities. The system integrates data from various sources, including satellite imagery, sensor networks, and ranger reports, to predict and identify high-risk areas for poaching.
The AI algorithms analyze patterns in poaching incidents, environmental factors, and ranger patrol data to generate real-time recommendations for optimal patrol routes and resource allocation. This has led to a significant reduction in elephant poaching within the targeted conservation areas.
Automated Coral Reef Monitoring in the Great Barrier Reef
The Great Barrier Reef in Australia faces numerous threats, including climate change, pollution, and disease outbreaks. Researchers have developed an AI-powered system to automate the monitoring and assessment of coral reef health.
The system uses underwater cameras and sensors to capture images and video of the reef, which are then analyzed by AI-based computer vision algorithms. These algorithms can identify and classify different coral species, assess the condition of the reef, and detect changes over time. The data collected is used to inform management decisions and guide conservation efforts.
Predicting Poaching Hotspots in South Africa
In the Kruger National Park in South Africa, park rangers and conservation authorities have partnered with AI researchers to develop a system for predicting and preventing poaching activities.
The AI-powered system combines data from various sources, including ranger patrols, sensor networks, and intelligence reports, to identify patterns and trends in poaching incidents. Using predictive modeling, the system can generate maps of high-risk poaching areas, allowing rangers to deploy targeted patrols and surveillance.
This approach has led to a significant decrease in the number of successful poaching incidents within the park, demonstrating the effectiveness of AI-driven decision support in wildlife conservation.
Monitoring Endangered Species in the Serengeti
The Serengeti National Park in Tanzania is home to a diverse array of wildlife, including many endangered species. Researchers have implemented an AI-powered system to monitor the populations and behaviors of these species.
The system utilizes a network of camera traps and sensor stations throughout the park, which capture images and video of the animals. AI-based computer vision algorithms are then used to identify individual animals, track their movements, and analyze their behaviors.
This data is used to inform conservation efforts, such as habitat management, anti-poaching patrols, and the development of targeted interventions to protect endangered species. The AI-powered monitoring system has provided valuable insights and helped to improve the overall management of the Serengeti ecosystem.
Challenges and Limitations of Using AI in Wildlife Conservation
Data Availability and Quality
One of the primary challenges in applying AI to wildlife conservation is the availability and quality of data. Comprehensive and reliable data on wildlife populations, habitats, and threats are often limited, particularly in remote or under-resourced regions.
- Incomplete or Biased Data: Many existing datasets may be incomplete, biased, or unevenly distributed, which can limit the accuracy and reliability of AI-based models.
- Data Collection Challenges: Collecting high-quality data on wildlife can be logistically and financially challenging, especially in remote or inaccessible areas.
- Privacy and Ethical Concerns: The use of AI-powered technologies, such as camera traps and drones, can raise concerns about privacy, data security, and the potential for misuse.
Technical Limitations and Computational Constraints
While AI has significant potential in wildlife conservation, there are also technical limitations and computational constraints that need to be addressed.
- Computational Power and Storage: The analysis of large-scale, high-resolution datasets, such as satellite imagery or audio recordings, can require significant computational resources and storage capacity, which may not be readily available in all conservation settings.
- Algorithm Limitations: Current AI algorithms may have limitations in areas such as species identification, behavior analysis, and predictive modeling, particularly for rare or elusive species.
- Interoperability and Data Integration: Effectively integrating and utilizing data from various sources, such as sensors, cameras, and databases, can be challenging and may require specialized technical expertise.
Interdisciplinary Collaboration and Capacity Building
Successful implementation of AI-powered solutions in wildlife conservation often requires close collaboration between domain experts, such as ecologists and conservation biologists, and AI/data science professionals.
- Bridging the Gap: Fostering effective communication and understanding between these diverse fields can be a significant challenge, as they may have different terminologies, priorities, and ways of working.
- Capacity Building: Building the necessary skills and knowledge within conservation organizations to effectively utilize AI technologies can be a time-consuming and resource-intensive process.
- Ethical and Responsible AI: Ensuring the ethical and responsible development and deployment of AI in wildlife conservation is crucial to maintain public trust and avoid unintended consequences.
Future Potential and Advancements in AI for Wildlife Conservation
Advancements in Computer Vision and Bioacoustics
Continued research and development in computer vision and bioacoustic technologies are expected to yield significant improvements in the accuracy and reliability of species identification, behavior monitoring, and habitat mapping.
- Improved Algorithms: Advancements in deep learning, transfer learning, and other AI techniques will enhance the ability of algorithms to recognize and classify species from visual and audio data.
- Multimodal Integration: Combining data from multiple sources, such as images, videos, and audio recordings, can provide a more comprehensive understanding of wildlife populations and their behaviors.
- Edge Computing and Embedded AI: The integration of AI capabilities directly into field devices and sensors can enable real-time analysis and decision-making at the point of data collection, reducing the need for centralized processing.
Advancements in Predictive Modeling and Decision Support
As the availability and quality of data improve, AI-powered predictive modeling and decision support systems are expected to become increasingly sophisticated and impactful in wildlife conservation.
- Integrated Threat Assessment: AI algorithms will be able to synthesize data from multiple sources to identify and assess a wide range of threats, such as poaching, habitat degradation, and climate change, enabling more comprehensive risk management.
- Adaptive Management Strategies: AI-powered systems will be able to continuously update their models and recommendations based on new data, allowing for more adaptive and responsive conservation strategies.
- Optimization and Scenario Planning: AI can be used to optimize resource allocation, personnel deployment, and conservation interventions, as well as to simulate and evaluate different conservation scenarios to support informed decision-making.
Advancements in Autonomous Systems and Robotics
Continued advancements in robotics and autonomous systems will likely lead to new and innovative applications in wildlife conservation.
- Autonomous Monitoring and Intervention: Drones, underwater robots, and other autonomous platforms will be able to conduct more extensive and frequent monitoring, as well as targeted interventions, with minimal human involvement.
- Improved Animal Welfare: AI-powered robotic systems can be designed to interact with wildlife in a way that minimizes stress and disturbance, supporting more humane and ethical conservation practices.
- Remote and Inaccessible Regions: Autonomous systems can access and operate in areas that are challenging or dangerous for human teams, expanding the reach and impact of conservation efforts.
Increased Collaboration and Interdisciplinary Approaches
Addressing the complex challenges in wildlife conservation will require even greater collaboration and integration of expertise from diverse fields, including ecology, computer science, engineering, social sciences, and policy.
- Transdisciplinary Partnerships: Fostering strong partnerships between conservation organizations, academic institutions, technology companies, and policymakers will be crucial for driving innovation and implementing effective AI-powered solutions.
- Citizen Science and Community Engagement: Engaging local communities and empowering them with AI-based tools and technologies can enhance data collection, conservation awareness, and collaborative decision-making.
- Ethical and Responsible AI Governance: Developing robust frameworks and guidelines for the ethical and responsible development and deployment of AI in wildlife conservation will be essential to ensure transparency, accountability, and public trust.
Conclusion
The integration of artificial intelligence in wildlife conservation holds immense promise, offering innovative solutions to pressing challenges and transforming the way we approach biodiversity monitoring, anti-poaching efforts, habitat management, and community engagement. As the field continues to evolve, the synergy between AI and conservation will become increasingly vital in our efforts to protect the world’s natural heritage.
By harnessing the power of AI, we can unlock new insights, make more informed decisions, and ultimately enhance the effectiveness and impact of our wildlife conservation initiatives. However, this journey is not without its challenges, and it will require close collaboration, capacity building, and a steadfast commitment to responsible and ethical AI development.
As we look to the future, the potential advancements in computer vision, bioacoustics, predictive modeling, autonomous systems, and interdisciplinary approaches hold the key to unlocking even greater breakthroughs in the field of wildlife conservation. By embracing these transformative technologies, we can pave the way for a more sustainable and resilient future, where the delicate balance of our planet’s ecosystems is preserved for generations to come.