In today’s rapidly evolving technological landscape, the convergence of Artificial Learning (AL) and the Internet of Things (IoT) is transforming the way devices communicate and operate. As AL algorithms empower IoT devices to process data intelligently, they enhance automation, efficiency, and decision-making capabilities across various industries. This comprehensive overview delves into the fundamentals of Artificial Learning, exploring its integration within IoT systems, the myriad benefits it brings to applications, and the key technologies driving this revolution. We will also examine real-world case studies, address the challenges and limitations inherent in this synergy, and look ahead to future trends that promise to redefine our connected world.
inxos.xyz will lead a thorough examination of this topic.
1. Definition and Fundamentals of Artificial Learning
Artificial Learning (AL) refers to the subset of machine learning that focuses on developing algorithms and models enabling machines to learn from data, improve over time, and make autonomous decisions. Unlike traditional programming, where explicit instructions are provided, AL systems leverage vast amounts of data to identify patterns, trends, and insights. This adaptive learning process allows devices to enhance their performance without human intervention.
The fundamentals of Artificial Learning encompass several key concepts, including supervised and unsupervised learning, reinforcement learning, and neural networks. Supervised learning involves training models on labeled datasets to predict outcomes, while unsupervised learning discovers hidden patterns in unlabeled data. Reinforcement learning, on the other hand, teaches systems to make decisions through trial and error, optimizing their strategies over time. As AL continues to advance, its ability to analyze and interpret complex data sets positions it as a vital component in the development and functionality of IoT devices, driving innovation across various sectors.

2. Integration of Artificial Learning in IoT Devices
The integration of Artificial Learning (AL) in Internet of Things (IoT) devices is a game changer, enabling these devices to process and analyze data in real-time. By embedding AL algorithms into IoT systems, devices can learn from their environments and make informed decisions autonomously. This seamless integration allows for smarter interactions, where IoT devices can adapt to user preferences, optimize performance, and predict maintenance needs.
For instance, smart home devices utilize AL to learn patterns in user behavior, adjusting heating, lighting, and security systems accordingly. In industrial settings, IoT sensors equipped with AL can monitor machinery performance, predicting failures before they occur and minimizing downtime. Additionally, wearable health devices analyze physiological data, providing personalized health insights and alerts.
The synergy between AL and IoT also enhances data management. By processing vast amounts of data locally, devices reduce the need for constant cloud communication, resulting in faster response times and improved privacy. As this integration continues to evolve, it paves the way for more innovative applications, making everyday objects smarter and more responsive, ultimately transforming how we interact with technology in our daily lives.
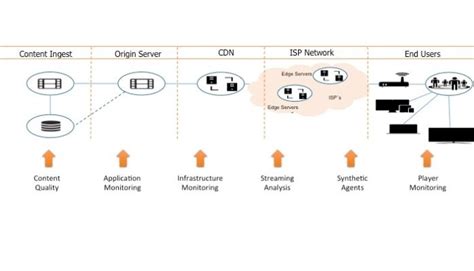
3. Benefits of Artificial Learning for IoT Applications
Artificial Learning (AL) offers numerous benefits for Internet of Things (IoT) applications, significantly enhancing their functionality and user experience. One of the primary advantages is improved decision-making. By analyzing vast amounts of real-time data, AL enables IoT devices to make informed choices, leading to optimized performance and greater efficiency. For example, smart appliances can adjust their operations based on usage patterns, resulting in energy savings and cost reductions.
Another key benefit is enhanced predictive capabilities. AL algorithms can identify patterns that help predict equipment failures or maintenance needs, allowing for proactive interventions. This predictive maintenance reduces downtime and operational costs in industrial settings.
Additionally, AL enhances personalization. IoT devices can learn individual user preferences, tailoring their functionality to meet specific needs. For instance, smart thermostats can adjust temperatures based on user habits, improving comfort and energy efficiency.
Furthermore, the integration of AL allows for real-time responses to changing conditions, making IoT systems more adaptable. Whether in smart cities, healthcare, or agriculture, AL empowers IoT applications to operate intelligently, fostering innovation and improving overall quality of life. As these technologies continue to evolve, their benefits will only expand, reshaping industries and daily experiences.
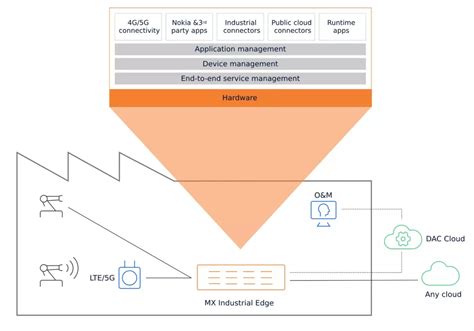
4. Key Technologies Driving Artificial Learning in IoT
Several key technologies are driving the integration of Artificial Learning (AL) in Internet of Things (IoT) applications, enabling smarter and more efficient devices. One of the foundational technologies is machine learning frameworks, such as TensorFlow and PyTorch, which facilitate the development of AL algorithms. These frameworks allow developers to create models that can analyze data, recognize patterns, and make predictions effectively.
Edge computing is another crucial technology, enabling data processing to occur closer to the source rather than relying solely on cloud computing. This reduces latency and bandwidth usage, allowing IoT devices to respond in real-time, which is essential for applications like autonomous vehicles and smart manufacturing.
Additionally, advanced sensors and connectivity technologies, such as 5G and LoRaWAN, enhance the data collection capabilities of IoT devices. These technologies enable devices to communicate efficiently and transmit large volumes of data quickly, which is vital for effective AL implementation.
Lastly, data analytics platforms are instrumental in transforming raw data into actionable insights. By leveraging big data analytics, organizations can extract valuable information from the vast amounts of data generated by IoT devices, driving the continuous improvement of AL algorithms and applications.
5. Case Studies of Artificial Learning in IoT Implementations
Numerous case studies illustrate the impactful implementations of Artificial Learning (AL) in Internet of Things (IoT) systems across various industries. One notable example is in smart agriculture, where AL-powered IoT sensors monitor soil conditions, weather patterns, and crop health. Farmers can receive real-time insights and recommendations for irrigation and fertilization, significantly increasing yields and resource efficiency.
In the healthcare sector, wearable devices equipped with AL analyze patient data to detect anomalies, such as irregular heartbeats. These devices alert healthcare providers promptly, enabling early intervention and improving patient outcomes.
Another compelling case is in smart cities, where AL algorithms optimize traffic flow by analyzing data from connected vehicles and infrastructure. This reduces congestion and enhances public transportation efficiency, leading to lower emissions and improved urban mobility.
In manufacturing, predictive maintenance systems utilize AL to monitor equipment performance and predict failures before they occur. This approach minimizes downtime and maintenance costs, streamlining operations.
These case studies highlight how AL, when integrated into IoT applications, drives innovation, enhances efficiency, and transforms operational practices across diverse sectors, ultimately leading to smarter and more sustainable solutions.
6. Challenges and Limitations of Artificial Learning in IoT
Despite the transformative potential of Artificial Learning (AL) in Internet of Things (IoT) applications, several challenges and limitations hinder its widespread adoption. One significant issue is data privacy and security. As IoT devices collect vast amounts of sensitive information, ensuring that this data is protected from breaches is paramount. Any compromise can lead to serious consequences for users and organizations alike.
Another challenge is the complexity of implementing AL algorithms. Developing effective models requires expertise in both machine learning and the specific domain of application, making it difficult for many organizations to leverage AL fully. Additionally, the quality of data is crucial; if the input data is flawed or biased, the AL models may produce inaccurate or misleading results.
Scalability is also a concern. As IoT networks expand, managing the increased data volume and ensuring consistent performance of AL systems can become overwhelming. Moreover, integration with existing infrastructure can pose technical hurdles, often requiring significant investment and resources.
Lastly, real-time processing demands may exceed the capabilities of some IoT devices, especially those with limited computing power. Addressing these challenges is essential for unlocking the full potential of AL in IoT applications and ensuring their effective implementation.
7. Future Trends and Predictions for Artificial Learning in IoT
Despite the transformative potential of Artificial Learning (AL) in Internet of Things (IoT) applications, several challenges and limitations hinder its widespread adoption. One significant issue is data privacy and security. As IoT devices collect vast amounts of sensitive information, ensuring that this data is protected from breaches is paramount. Any compromise can lead to serious consequences for users and organizations alike.
Another challenge is the complexity of implementing AL algorithms. Developing effective models requires expertise in both machine learning and the specific domain of application, making it difficult for many organizations to leverage AL fully. Additionally, the quality of data is crucial; if the input data is flawed or biased, the AL models may produce inaccurate or misleading results.
Scalability is also a concern. As IoT networks expand, managing the increased data volume and ensuring consistent performance of AL systems can become overwhelming. Moreover, integration with existing infrastructure can pose technical hurdles, often requiring significant investment and resources.
Lastly, real-time processing demands may exceed the capabilities of some IoT devices, especially those with limited computing power. Addressing these challenges is essential for unlocking the full potential of AL in IoT applications and ensuring their effective implementation.
In conclusion, the integration of Artificial Learning in Internet of Things applications is reshaping industries by enhancing decision-making, personalization, and efficiency. While challenges such as data privacy, implementation complexity, and scalability remain, the benefits are substantial. As technology continues to evolve, addressing these hurdles will be crucial in unlocking the full potential of AL in IoT, paving the way for smarter, more connected environments that improve quality of life and operational effectiveness.
inxos.xyz