Artificial intelligence (AI) has emerged as a powerful and transformative technology, revolutionizing industries and shaping our daily lives. From personalized recommendations on streaming services to automated decision-making processes in healthcare and finance, AI has become ubiquitous. However, this rapid advancement has also brought to light a darker truth – the potential for algorithmic bias. Bias in AI refers to the systematic and unfair treatment of individuals or groups based on their characteristics, perpetuated by algorithms built on biased data and design decisions. This article will delve into the nuances of bias in AI and explore efforts being made to address it.
Understanding Bias in AI and Machine Learning
To effectively address bias in AI, it is crucial to understand its origins. Algorithmic bias typically arises from two main sources: data used for training AI models and the inherent biases of their designers.
Data Bias: The Feed of the Machine
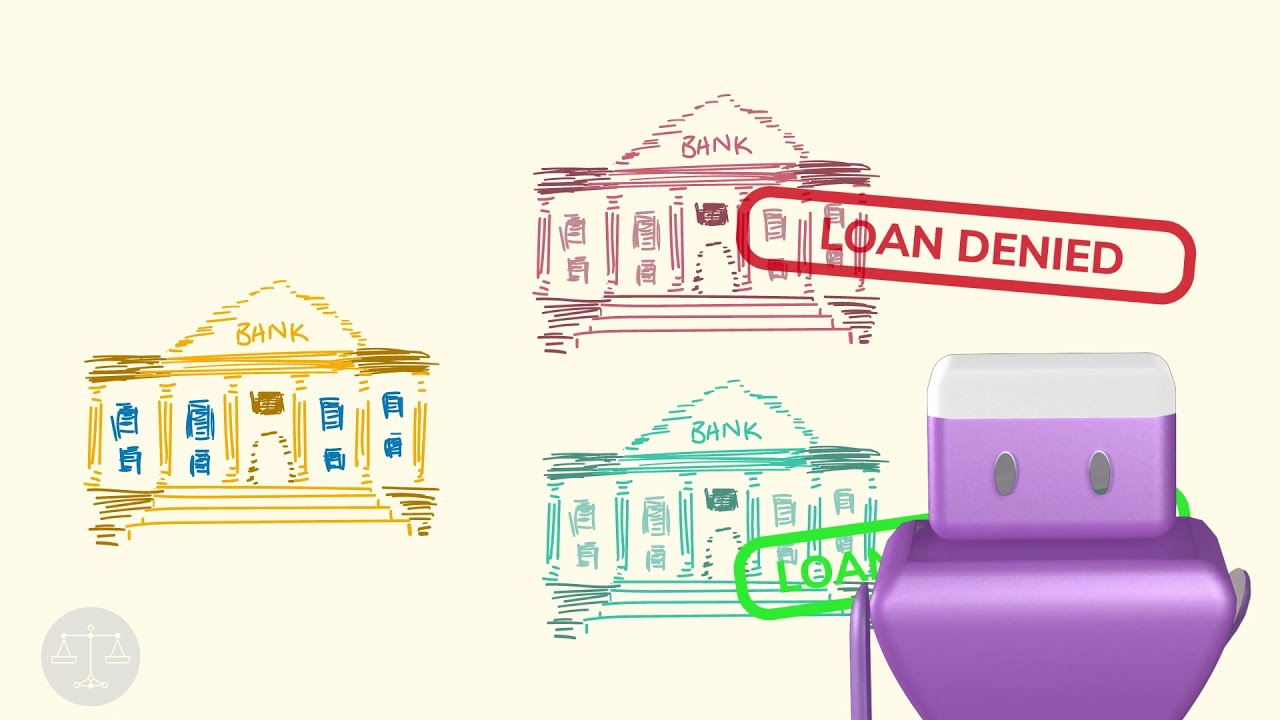
AI algorithms are trained on vast datasets, which serve as their learning material. If these datasets are inherently biased, reflecting existing societal prejudices, the resulting algorithms will inevitably inherit these biases. For instance, if a facial recognition system is trained primarily on images of light-skinned individuals, it may struggle to accurately identify darker-skinned faces, leading to discriminatory outcomes.
Historical Data: A Legacy of Prejudice
Many datasets employed for AI training are derived from historical records, which are often riddled with discriminatory practices and societal norms. These records reflect the biases and prejudices of the time, and as a result, AI models trained on such data can perpetuate these biases in their decision-making processes. For example, a hiring algorithm may be biased against women if it is trained on historical data that shows a disproportionate number of men in certain job positions.
Unbalanced Data: Reinforcing Stereotypes
Another source of data bias is unbalanced datasets, where certain groups are underrepresented or excluded altogether. This can lead to inaccurate and biased predictions, as the algorithm has not been exposed to a diverse range of data. For instance, if an AI system is trained on data from a specific geographical region, it may struggle to make accurate predictions for individuals from different regions.
Design Bias: The Influence of Human Prejudice

The second source of algorithmic bias is the inherent biases of the designers themselves. AI systems are built by human programmers who, like any other human, can bring their own biases, conscious or unconscious, into their work. These biases can manifest in various ways, from the selection of data used to train the models to the design of algorithms and the parameters set for decision-making.
Objectivity vs. Subjectivity: The Illusion of Fairness
One common misconception is that AI systems are inherently objective, free from human biases. However, this is not the case – humans are the ones designing and programming these systems, and they bring their own subjectivities into the process. Even seemingly neutral data can carry biases due to the context in which it was collected or the way it was labeled. This can result in biased decisions and perpetuate existing inequalities.
Lack of Diversity in Tech: A Saturated Industry
The tech industry is notorious for its lack of diversity, particularly in positions of power and influence. This homogeneity can result in a narrow perspective and limited understanding of the diverse needs and experiences of different groups. As a result, the biases of these individuals can become embedded in the design and development of AI systems.
Impact of Bias in AI and Machine Learning
The consequences of bias in AI can be far-reaching and have significant implications for society. Here are some of the major impacts of bias in AI and machine learning:
Amplification of Inequalities
Bias in AI can exacerbate and reinforce existing societal inequalities. If an AI system is biased against a particular group, it can lead to discriminatory outcomes, further marginalizing and disadvantaging these individuals. For instance, if a loan approval algorithm discriminates against certain ethnic groups, it can perpetuate the wealth gap and deny opportunities to those in need.
Reproduction of Prejudice
AI systems trained on biased data can also reproduce and perpetuate discriminatory practices and stereotypes. For example, a recruitment algorithm may learn to prefer candidates from a particular age group or educational background, reflecting the biases of the data used for training.
Loss of Trust and Accountability
When AI systems make decisions that are biased and unfair, it can erode trust in these technologies and their creators. This can have significant consequences for industries that rely heavily on AI and machine learning, such as healthcare and finance. Additionally, it can also hinder accountability, as it becomes challenging to assign responsibility for biased outcomes when they are produced by seemingly neutral algorithms.
Current Efforts to Address Bias
The recognition of bias in AI has sparked efforts to address and mitigate its impact. Here are some of the current initiatives being undertaken:
Diverse and Representative Data Collection
To tackle data bias, there is a growing emphasis on collecting diverse and representative datasets. This involves actively seeking out data from different sources and ensuring that it accurately reflects the diversity of the population.
Algorithmic Audits and Transparency
Algorithmic audits involve reviewing the decision-making processes of AI systems to identify and correct any potential biases. This can be done through robust testing and continuous monitoring of AI systems. Additionally, transparency in the development and deployment of AI can help hold developers accountable and increase trust in these technologies.
Diversity and Inclusion in Tech
Efforts are being made to improve diversity and inclusion in the tech industry, which can help combat design bias. Companies are implementing programs to increase diversity in their hiring practices and promoting an inclusive work culture to foster a diverse perspective.
Challenges in Addressing Bias
Despite the efforts being made, addressing bias in AI is not without its challenges. Here are some of the key obstacles in this endeavor:
Lack of Diversity in Data
One of the biggest challenges in addressing bias is the lack of diverse and representative data. This can make it difficult to identify and mitigate bias in AI systems adequately.
Complex and Evolving Nature of Bias
Bias in AI is a complex and constantly evolving issue, making it challenging to develop a one-size-fits-all solution. As societal norms and attitudes continue to change, so too does the nature of bias, requiring ongoing efforts to address it effectively.
Resistance to Change
Many companies and individuals may be hesitant to address bias in their AI systems due to concerns about the cost and time involved in making changes. Additionally, there may also be resistance from those who benefit from biased outcomes, making it challenging to implement meaningful change.
Ethical Considerations
The presence of bias in AI raises significant ethical considerations regarding the use and development of these technologies. Here are some key ethical considerations to keep in mind:
Fairness and Non-Discrimination
AI systems should be built and deployed with fairness and non-discrimination in mind. This means considering and addressing the potential biases that may arise from data and design decisions.
Transparency and Explainability
Transparency and explainability are crucial for building trust and accountability in AI systems. Users should be able to understand how a decision was made by an AI system and have access to information about the data used and any potential biases.
Responsibility and Accountability
As AI becomes more pervasive in our lives, it is essential to establish clear lines of responsibility and accountability for the decisions made by these systems. It should be clear who is responsible for addressing any potential biases and ensuring fair outcomes.
Future Directions and Solutions
Addressing bias in AI requires a multifaceted approach involving collaboration and ongoing efforts from various stakeholders. Here are some of the potential solutions and future directions for addressing bias in AI:
Diverse Teams and Perspectives
Creating diverse teams with individuals from different backgrounds can help mitigate bias in AI. These diverse perspectives can bring a more nuanced understanding of potential biases and promote inclusive decision-making.
Regular Audits and Evaluations
Regular audits and evaluations of AI systems can help detect and address any potential biases early on. With the ever-evolving nature of bias, ongoing evaluation and monitoring are crucial for ensuring fair outcomes.
Ethical Guidelines and Standards
The development of ethical guidelines and standards specific to AI can help guide the responsible use and deployment of these technologies. These guidelines should address issues such as fairness, transparency, and accountability.
Conclusion
As AI continues to become more integrated into our lives, it is essential to address the issue of bias to ensure equitable and fair outcomes for all. Efforts are underway to mitigate bias in AI, but it requires ongoing collaboration and a commitment to diversity and inclusivity in the development and deployment of these technologies. By working together and remaining vigilant, we can navigate the ethical landscape of AI and create a more just and fair society for all.