Climate modeling and prediction are critical for understanding and addressing the complex challenges posed by climate change. As the impacts of climate change become increasingly severe, the need for accurate and reliable climate forecasting has never been greater. In this blog post, we will explore the role of artificial intelligence (AI) in enhancing climate modeling and prediction capabilities.
Introduction
Climate modeling and prediction are essential for a wide range of applications, from disaster preparedness and resource management to urban planning and agriculture. However, the complexity of Earth’s climate system, with its vast array of interacting physical, chemical, and biological processes, poses significant challenges for traditional modeling approaches. This is where AI technology can play a transformative role, offering new and powerful tools for understanding and predicting climate patterns.
Importance of Climate Modeling and Prediction
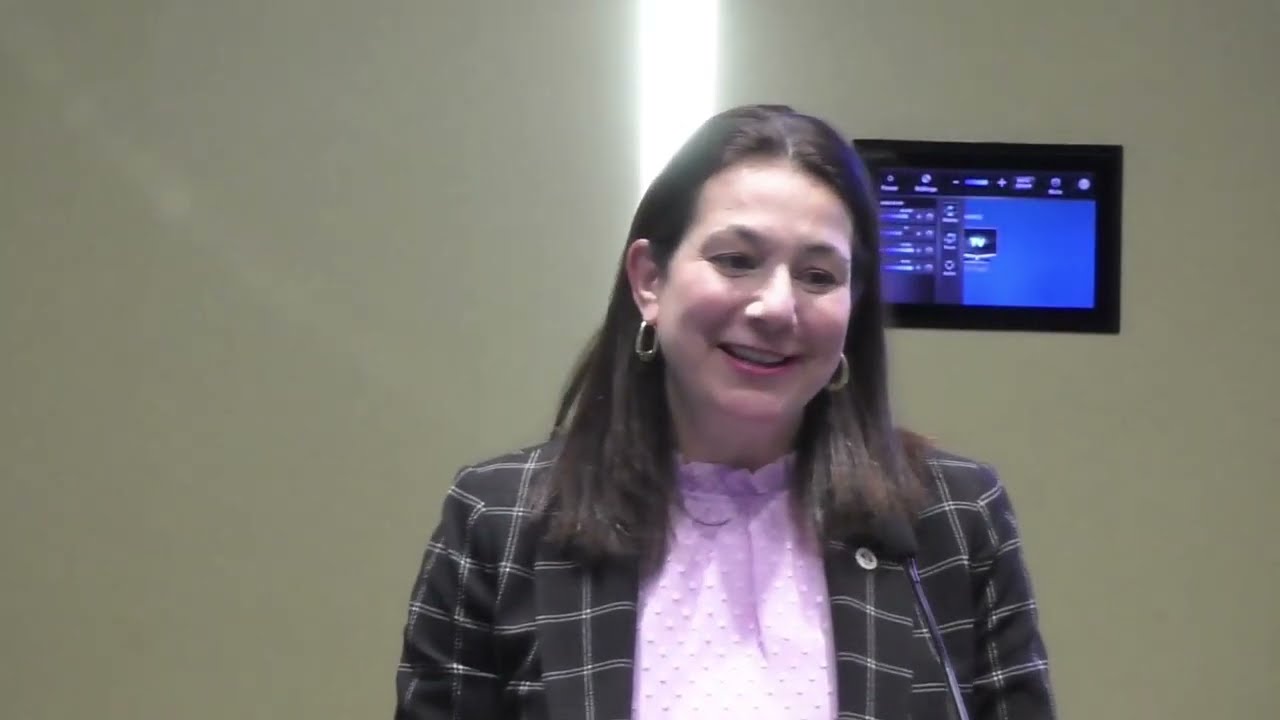
Climate modeling and prediction are crucial for several reasons:
- Forecasting and Preparedness: Accurate climate predictions enable better planning and preparedness for extreme weather events, such as hurricanes, droughts, and heatwaves, helping to mitigate their impact on communities and infrastructure.
- Resource Management: Climate models can inform decision-making around the management of natural resources, such as water, agriculture, and energy, ensuring sustainable practices and efficient allocation of resources.
- Adaptation and Mitigation Strategies: Climate models help policymakers and stakeholders develop effective strategies for adapting to the changing climate and mitigating its long-term effects.
- Scientific Understanding: Climate models enhance our understanding of the complex Earth system, enabling researchers to study the drivers and impacts of climate change, and to test hypotheses about the future of our planet.
Overview of AI Technology
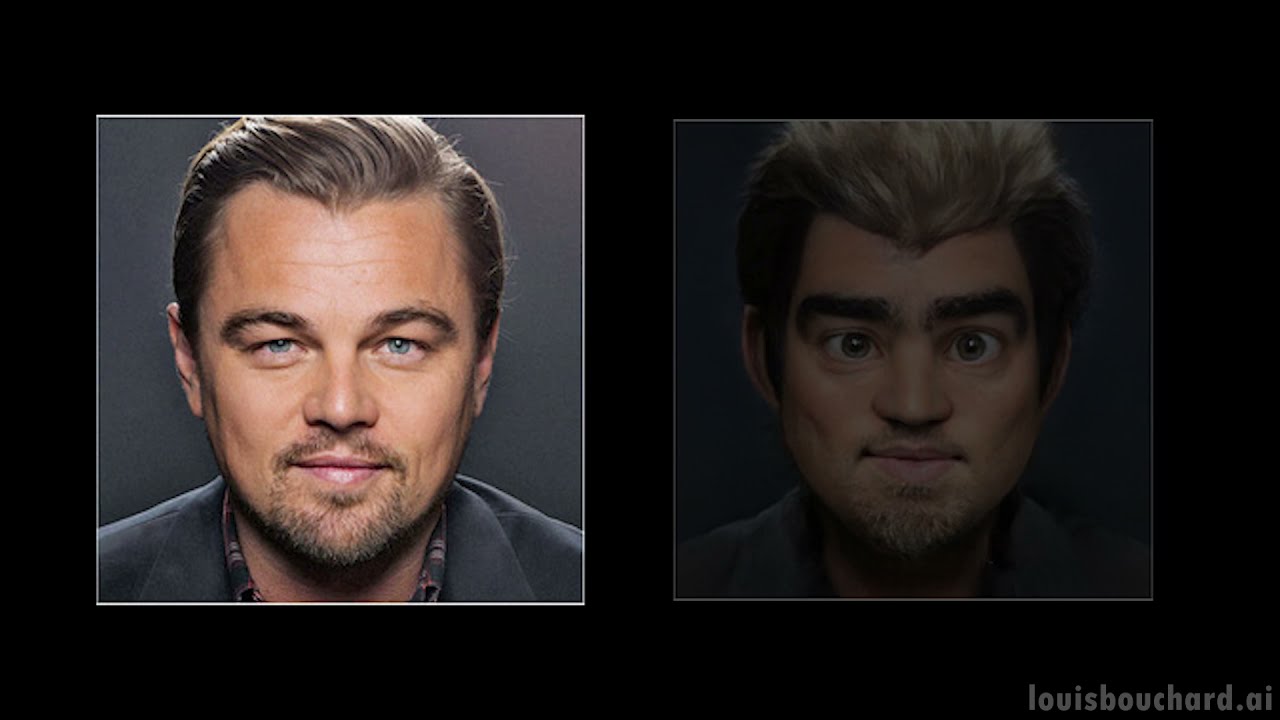
Artificial intelligence is a rapidly advancing field that encompasses a wide range of techniques, including machine learning, deep learning, and natural language processing. These AI techniques have proven to be highly effective in a variety of domains, from image recognition to language translation, and are increasingly being applied to the realm of climate science.
Machine Learning
Machine learning algorithms can be trained on vast datasets of climate observations and simulations, enabling them to identify patterns, recognize trends, and make predictions about future climate conditions. These algorithms can learn from historical data and adapt to new information, making them highly versatile and capable of handling the complex, nonlinear relationships inherent in climate systems.
Deep Learning
Deep learning, a subset of machine learning, utilizes artificial neural networks with multiple hidden layers to extract increasingly complex features from data. This approach has shown remarkable success in modeling and predicting climate phenomena, such as the formation and behavior of hurricanes, the evolution of sea ice, and the patterns of precipitation and temperature.
Natural Language Processing
Natural language processing (NLP) can be used to extract and analyze climate-related information from a wide range of sources, including scientific literature, news articles, and social media. This can help researchers and decision-makers to stay informed about the latest developments in climate science and to identify emerging trends and issues.
Applications of AI in Climate Modeling
AI is being applied to climate modeling and prediction in a variety of ways, including:
1. Improved Climate Simulations
AI-powered climate models can simulate the complex interactions between the atmosphere, oceans, land, and biosphere with greater accuracy and efficiency than traditional models. These models can incorporate a wider range of data sources, from satellite imagery to in-situ observations, to create more comprehensive and realistic representations of the climate system.
Enhancing Model Parameterization
Climate models rely on parameterizations, which are simplified representations of complex physical processes, such as cloud formation and convection. AI techniques can be used to improve these parameterizations, allowing for more accurate simulations of these processes and reducing the uncertainties associated with climate projections.
Reducing Computational Complexity
Climate models often require vast computational resources to run, as they must simulate the Earth’s climate system at high spatial and temporal resolutions. AI-based approaches can help reduce the computational complexity of these models, making them more efficient and accessible to a wider range of researchers and institutions.
Improving Data Assimilation
Data assimilation is the process of incorporating observational data into climate models to improve their accuracy. AI can be used to enhance data assimilation techniques, allowing for more efficient and effective integration of diverse data sources, such as satellite observations, ground-based measurements, and climate model outputs.
2. Extreme Event Prediction
AI-based models have shown promising results in predicting the occurrence, intensity, and impacts of extreme weather events, such as hurricanes, heatwaves, and droughts. These models can leverage a wide range of data sources, including meteorological observations, satellite imagery, and climate model simulations, to identify patterns and make more accurate predictions.
Anticipating Hurricane Tracks and Intensities
AI can be used to improve the prediction of hurricane tracks and intensities, which is crucial for emergency planning and response efforts. Machine learning algorithms can analyze historical hurricane data, along with real-time observations and forecasts, to generate more accurate and reliable hurricane predictions.
Forecasting Heatwaves and Droughts
AI-based models can also be used to predict the onset, duration, and severity of heatwaves and droughts, which are becoming increasingly frequent and intense due to climate change. These predictions can inform decision-making around water resource management, agricultural planning, and public health interventions.
Enhancing Early Warning Systems
AI-powered early warning systems can integrate various data sources, such as weather forecasts, satellite observations, and social media, to provide timely and accurate alerts about impending extreme weather events. These systems can help communities and emergency responders prepare and mitigate the impacts of these events.
3. Climate Impact Assessment and Adaptation
AI can play a significant role in assessing the impacts of climate change and informing adaptation strategies. AI-based models can simulate the effects of climate change on various sectors, such as agriculture, water resources, and infrastructure, and help identify the most vulnerable regions and populations.
Modeling Climate Impacts on Agriculture
AI can be used to model the impacts of climate change on agricultural productivity, including changes in crop yields, pest and disease outbreaks, and water availability. This information can guide farmers, policymakers, and stakeholders in developing more resilient and sustainable agricultural practices.
Assessing Risks to Infrastructure and Ecosystems
AI-based models can also assess the risks posed by climate change to critical infrastructure, such as transportation networks, energy systems, and coastal communities. Additionally, these models can help evaluate the impacts on natural ecosystems, informing conservation and restoration efforts.
Supporting Adaptation Planning
AI can support the development of climate adaptation strategies by identifying the most effective and cost-efficient measures, such as flood protection, drought-resistant agriculture, and ecosystem restoration. These insights can inform decision-making and help communities become more resilient to the effects of climate change.
Challenges and Limitations
While the application of AI in climate modeling and prediction offers significant potential, there are also several challenges and limitations that must be addressed:
Data Availability and Quality
The success of AI-based climate models depends on the availability and quality of the underlying data. Gaps in observational data, especially in regions with limited monitoring infrastructure, can hinder the effectiveness of these models.
Model Complexity and Interpretability
The complexity of AI-based climate models can make it challenging to understand the underlying mechanisms and causal relationships. Ensuring the interpretability of these models is crucial for building trust and facilitating their integration into decision-making processes.
Computational Resources
Running advanced AI-based climate models requires significant computational resources, which may not be readily available to all researchers and institutions. This can limit the accessibility and scalability of these technologies.
Validation and Uncertainty Quantification
Validating the performance of AI-based climate models and quantifying their uncertainties is essential for ensuring the reliability and trustworthiness of their predictions. Developing robust validation frameworks and uncertainty quantification methods is an ongoing challenge.
Ethical Considerations
The application of AI in climate modeling and prediction raises ethical questions, such as the potential for biased or discriminatory outcomes, the transparency of decision-making processes, and the responsible use of technology in addressing climate change.
Future Developments and Possibilities
As the field of AI continues to evolve, the potential applications in climate modeling and prediction are vast and exciting. Here are some of the future developments and possibilities:
Hybrid Modeling Approaches
Combining AI-based techniques with traditional physical-based climate models can lead to the development of hybrid modeling approaches that leverage the strengths of both approaches, resulting in more accurate and robust climate simulations.
Automated Model Discovery and Design
AI could be used to automate the process of discovering and designing new climate models, exploring a wider range of modeling approaches and accelerating the pace of scientific progress in this field.
Integrated Decision Support Systems
AI-powered decision support systems could integrate climate modeling, impact assessment, and adaptation planning, providing policymakers and stakeholders with comprehensive, data-driven insights to guide climate-related decision-making.
Personalized Climate Services
AI could enable the development of personalized climate services, tailored to the specific needs and preferences of individual users, such as farmers, urban planners, or public health officials.
Democratizing Climate Science
By making AI-based climate modeling and prediction tools more accessible and user-friendly, the application of these technologies could be democratized, empowering a wider range of stakeholders to engage with and contribute to climate science.
Conclusion
The integration of AI technology into climate modeling and prediction offers immense potential to enhance our understanding of the Earth’s complex climate system and to develop more accurate and reliable forecasts. As we face the growing challenges of climate change, the application of AI in this domain can help inform critical decision-making, support adaptation and mitigation efforts, and ultimately contribute to a more sustainable future for our planet.