The growing demand for energy, coupled with the increasing complexity of energy systems, has made efficient energy management a critical challenge for both individuals and organizations. Fortunately, the advent of Artificial Intelligence (AI) has presented new opportunities to address these challenges. AI-powered energy management solutions have the potential to revolutionize the way we generate, distribute, and consume energy, leading to significant improvements in energy efficiency, cost savings, and environmental sustainability.
Introduction to AI in energy management
Artificial Intelligence is a field of computer science that focuses on the development of systems and algorithms that can perform tasks typically requiring human intelligence, such as problem-solving, decision-making, and pattern recognition. In the context of energy management, AI can be leveraged to optimize various aspects of energy systems, including generation, distribution, and consumption.
Understanding AI’s role in energy management
AI can play a crucial role in energy management by providing intelligent solutions for a wide range of tasks, such as:
- Predictive analytics: AI-powered models can analyze historical data and current trends to predict future energy demand, generation, and grid conditions, enabling more effective planning and decision-making.
- Real-time optimization: AI algorithms can continuously monitor and adjust energy systems in real-time, optimizing energy usage and distribution to improve efficiency and reduce costs.
- Anomaly detection: AI can detect and identify unusual patterns or anomalies in energy systems, allowing for early intervention and prevention of potential issues.
- Automated decision-making: AI systems can make complex, data-driven decisions about energy management, such as when to switch between different energy sources or how to allocate resources.
- Intelligent control systems: AI-powered control systems can integrate and coordinate various components of an energy system, such as renewable energy sources, storage, and consumption, to optimize overall performance.
The evolution of AI in energy management
The application of AI in energy management has evolved significantly in recent years, driven by advancements in machine learning, data analytics, and computing power. As the technology continues to mature, AI-based energy management solutions are becoming increasingly sophisticated and effective, addressing a wide range of challenges faced by the energy industry.
Importance of energy optimization
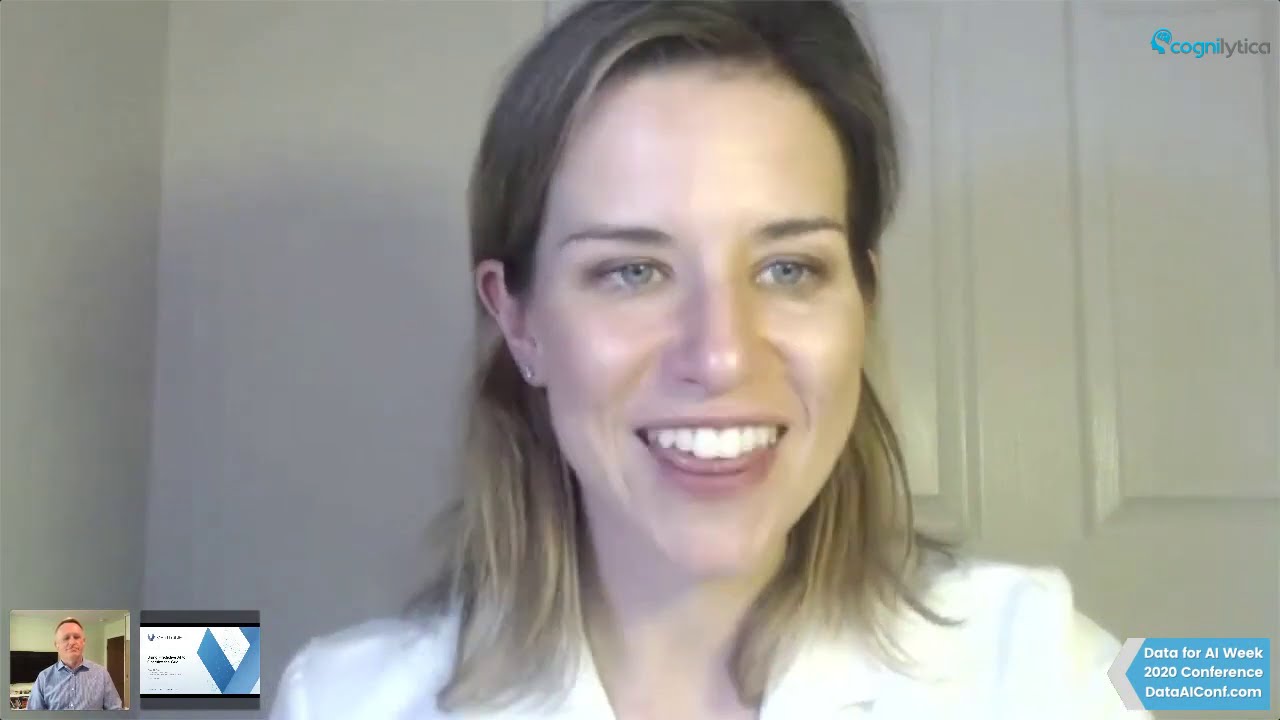
Energy optimization is crucial for both individual and organizational sustainability, as it can lead to significant cost savings, reduced environmental impact, and improved energy security.
Cost savings
Effective energy optimization can result in substantial cost savings for both households and businesses. By reducing energy consumption and improving efficiency, organizations can lower their operational expenses, while individuals can save on their utility bills.
Environmental sustainability
The energy sector is a significant contributor to greenhouse gas emissions and environmental degradation. By optimizing energy usage and shifting towards renewable and clean energy sources, AI-powered solutions can play a crucial role in reducing the environmental impact of energy consumption.
Energy security and reliability
Optimizing energy management can enhance the reliability and resilience of energy systems, helping to mitigate the risk of power outages and ensure a stable and secure energy supply.
Applications of AI in energy management
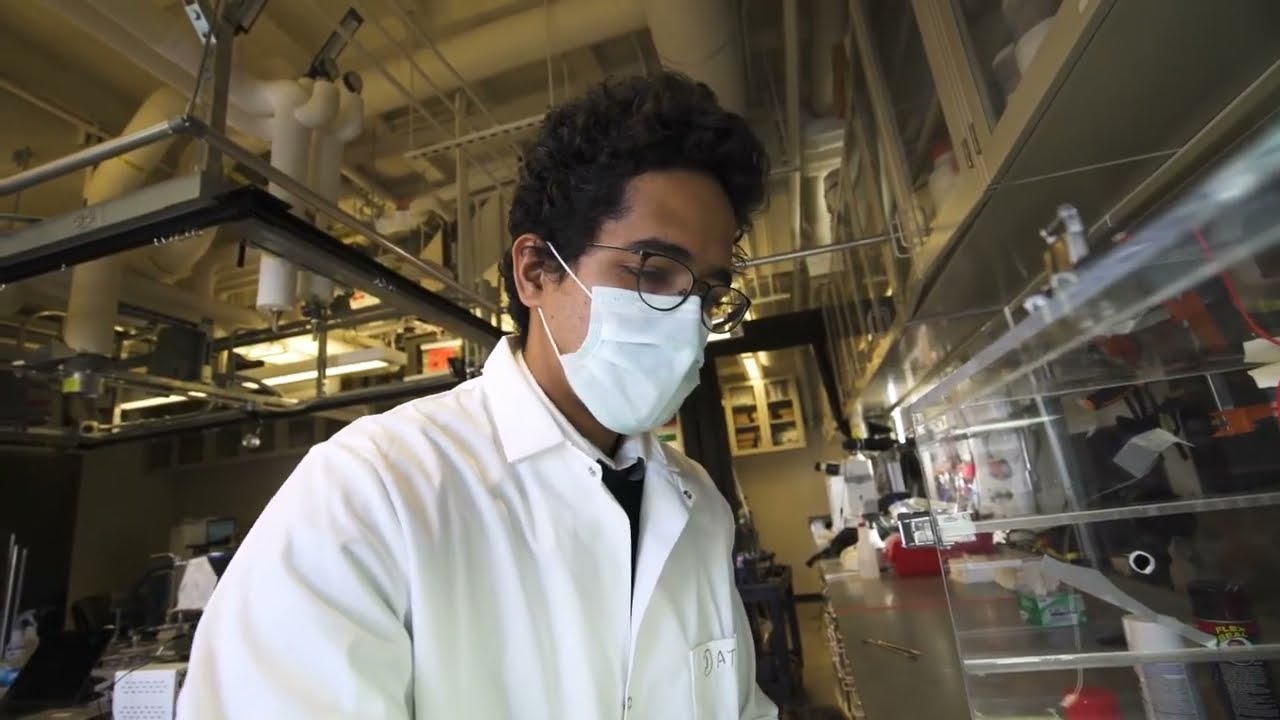
AI has a wide range of applications in the energy management domain, spanning generation, distribution, and consumption.
AI-powered renewable energy optimization
AI can be used to optimize the performance of renewable energy systems, such as solar and wind power, by:
- Forecasting energy generation based on weather patterns and historical data
- Adjusting the output of renewable energy sources to match real-time demand
- Coordinating the integration of renewable energy with traditional energy sources
Smart grid management and optimization
AI can play a crucial role in the development and optimization of smart grids, which are digitally-enabled electricity networks that can intelligently manage the flow of energy. AI-powered smart grid solutions can:
- Optimize grid operations and load balancing
- Predict and mitigate grid congestion and outages
- Facilitate the integration of distributed energy resources
- Enhance energy distribution and transmission efficiency
Intelligent building energy management
AI can be applied to optimize energy usage in buildings, both residential and commercial, by:
- Analyzing occupancy patterns and user preferences to adjust heating, cooling, and lighting
- Predicting energy demand and optimizing building systems accordingly
- Identifying and addressing energy inefficiencies within the building
- Integrating building energy management with broader energy grid optimization
Transportation and electric vehicle (EV) energy optimization
AI can be leveraged to optimize energy usage in the transportation sector, particularly in the context of electric vehicles (EVs), by:
- Predicting EV charging demand and coordinating charging schedules
- Optimizing the routing and scheduling of electric fleet vehicles
- Balancing EV charging with grid-level energy management
Industrial energy optimization
AI can be applied to optimize energy usage in industrial settings, such as manufacturing plants and factories, by:
- Identifying and addressing energy inefficiencies in industrial processes
- Optimizing the scheduling and coordination of energy-intensive equipment
- Integrating industrial energy management with broader grid-level optimization
Household and consumer energy optimization
AI-powered solutions can also be used to optimize energy usage at the household and individual consumer level, by:
- Providing personalized recommendations for energy-saving behaviors
- Automatically adjusting home appliances and systems based on user preferences and energy prices
- Monitoring and reporting on energy consumption to encourage conservation
Case studies of successful AI implementations
To illustrate the real-world impact of AI in energy management, let’s explore a few case studies of successful AI implementations:
Case Study 1: AI-powered renewable energy optimization at a wind farm
A large wind farm operator in the United States implemented an AI-based system to optimize the performance of its wind turbines. The system utilized machine learning algorithms to:
- Analyze historical wind and weather data to accurately predict energy generation
- Adjust the pitch and yaw of the turbines in real-time to maximize energy output
- Identify and address potential maintenance issues before they occurred
The results were remarkable, with a 5-7% increase in energy generation and a 15% reduction in maintenance costs.
Case Study 2: Smart grid optimization in a major metropolitan area
A major city in Europe implemented an AI-powered smart grid system to manage its electricity network more efficiently. The system:
- Leveraged predictive analytics to forecast energy demand and generation
- Automatically adjusted the flow of electricity across the grid to minimize losses and optimize distribution
- Detected and addressed grid anomalies and outages in real-time
The implementation of the AI-driven smart grid system resulted in a 12% reduction in energy losses, a 20% improvement in grid reliability, and significant cost savings for the city and its residents.
Case Study 3: AI-enabled building energy management in a commercial office complex
A large commercial office building in Asia adopted an AI-powered energy management system to optimize its energy usage. The system:
- Analyzed occupancy patterns and user preferences to adjust heating, cooling, and lighting accordingly
- Identified and addressed energy inefficiencies within the building’s systems
- Integrated with the local energy grid to coordinate energy consumption and minimize peak demand charges
The results were impressive, with a 25% reduction in the building’s energy consumption and a 30% decrease in energy costs.
Challenges and future trends in AI for energy optimization
While the potential of AI in energy management is significant, there are also several challenges and emerging trends that must be considered.
Challenges
- Data availability and quality: Effective AI-powered energy management solutions rely on access to large, high-quality datasets. Ensuring the availability and integrity of this data can be a significant challenge, particularly in older or less technologically advanced energy systems.
- Cybersecurity and data privacy: As AI-powered energy management systems become more prevalent, the risk of cyber threats and data breaches increases. Robust cybersecurity measures and data privacy protocols are essential to protect critical energy infrastructure and consumer information.
- Regulatory and policy barriers: The energy industry is often heavily regulated, and the adoption of AI-based solutions may be hindered by outdated policies or regulatory frameworks that do not accommodate this emerging technology.
- Integration and interoperability: Integrating AI-powered energy management solutions with existing infrastructure and systems can be a complex and challenging task, requiring careful planning and coordination.
- Explainability and trust: For AI-powered energy management solutions to be widely accepted, users must be able to understand and trust the decision-making process of the AI systems. Ensuring transparency and explainability is a crucial challenge.
Future trends
- Increased integration of AI and renewable energy: As the world transitions to a more sustainable energy mix, the integration of AI with renewable energy sources, such as solar and wind, will become increasingly important to optimize their performance and integration into the grid.
- Distributed and decentralized energy management: The rise of decentralized energy systems, such as microgrids and peer-to-peer energy trading platforms, will drive the development of AI-powered solutions that can manage and optimize these distributed energy resources.
- Personalized and adaptive energy management: AI-powered energy management solutions will become more personalized and adaptive, catering to the unique needs and preferences of individual consumers and buildings.
- Predictive maintenance and asset optimization: AI will play a crucial role in predictive maintenance and asset optimization, helping to identify potential issues in energy infrastructure and optimize the performance and lifespan of critical components.
- Increased focus on sustainability and environmental impact: As the imperative to address climate change and environmental concerns grows, AI-powered energy management solutions will need to prioritize sustainability and minimize the environmental impact of energy systems.
Conclusion
Artificial Intelligence has the potential to revolutionize the way we manage and optimize energy systems, leading to significant improvements in efficiency, cost savings, and environmental sustainability. By leveraging AI’s capabilities in predictive analytics, real-time optimization, and intelligent control, energy providers and consumers can unlock new opportunities to address the complex challenges faced by the energy industry.
As the technology continues to evolve and become more widely adopted, we can expect to see even greater advancements in AI-powered energy management solutions, driving us towards a more sustainable and resilient energy future. However, to fully realize the benefits of AI in energy management, it will be essential to overcome the challenges related to data, cybersecurity, regulation, and integration, while embracing the emerging trends that will shape the energy landscape in the years to come.