As the world grapples with the pressing need to transition to sustainable energy sources, the role of artificial intelligence (AI) in renewable energy has become increasingly crucial. AI-powered solutions have the potential to revolutionize the way we harness, manage, and optimize clean energy resources, paving the way for a more efficient and sustainable future.
Overview of AI in Renewable Energy
Understanding the Integration of AI and Renewable Energy
The integration of AI and renewable energy is a symbiotic relationship, where AI algorithms and technologies are employed to enhance the performance, reliability, and efficiency of renewable energy systems. From wind and solar power to hydroelectric and geothermal energy, AI is being used to optimize various aspects of these clean energy solutions.
The Emergence of AI-Powered Renewable Energy Systems
In recent years, the advancement of AI technology has led to the emergence of sophisticated renewable energy systems that leverage machine learning, deep learning, and other AI-based techniques. These systems are designed to gather, analyze, and act upon real-time data, enabling intelligent decision-making and automated optimization to maximize energy generation and distribution.
The Role of AI in Different Renewable Energy Sectors
AI has found applications across various renewable energy sectors, including:
- Solar Energy: AI is used to predict solar irradiation, optimize panel orientation, and enhance the performance of photovoltaic systems.
- Wind Energy: AI algorithms are employed to forecast wind patterns, optimize turbine blade pitch, and improve overall wind farm efficiency.
- Hydroelectric Power: AI is utilized to predict water flow, manage reservoir levels, and optimize hydroelectric power generation.
- Geothermal Energy: AI-powered systems are used to analyze geological data, optimize well placement, and enhance the extraction of geothermal energy.
Benefits of AI in Renewable Energy
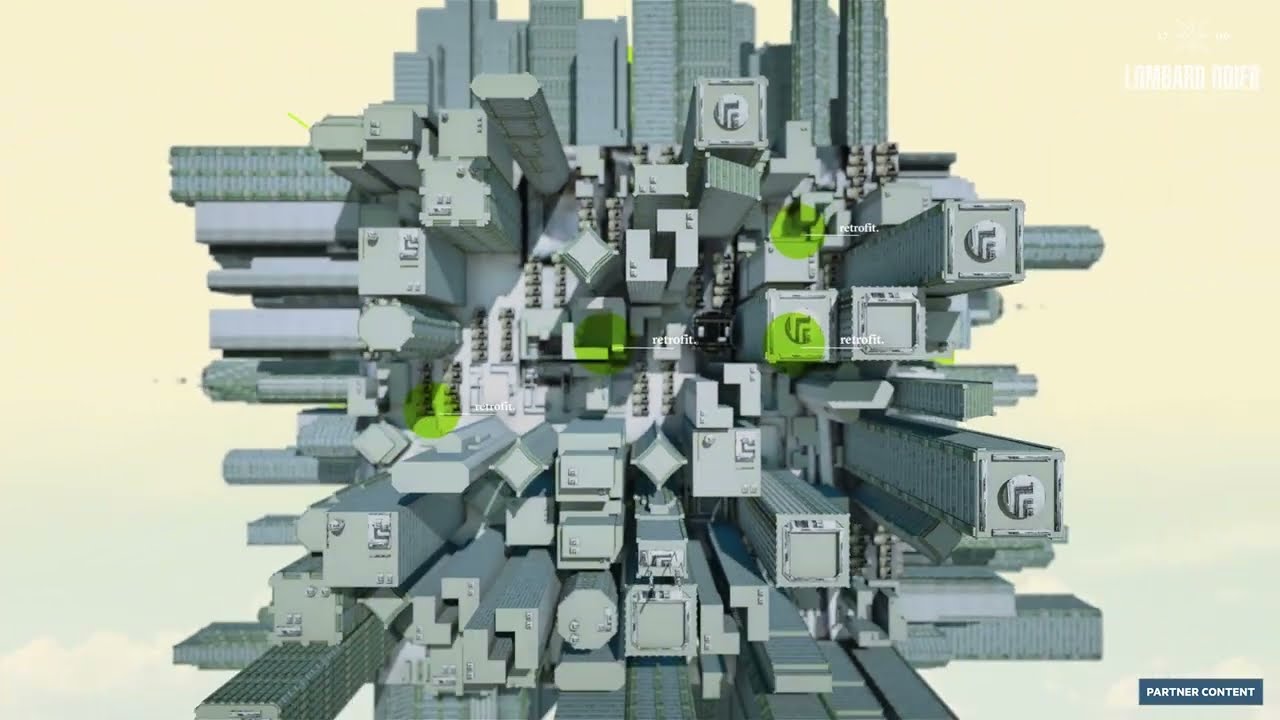
Improved Efficiency and Performance
One of the primary benefits of AI in renewable energy is its ability to enhance system efficiency and performance. AI algorithms can analyze vast amounts of data, identify patterns, and make real-time adjustments to optimize energy generation, storage, and distribution. This can lead to increased energy output, reduced waste, and improved overall system performance.
Enhanced Predictive Capabilities
AI-powered predictive analytics play a crucial role in renewable energy systems. By leveraging historical data, weather forecasts, and other relevant information, AI can accurately predict factors such as solar irradiation, wind patterns, and water flow, enabling better planning and resource management.
Automated and Intelligent Decision-Making
AI-driven renewable energy systems are capable of making autonomous decisions based on real-time data and predetermined optimization goals. This automation can lead to faster response times, reduced human intervention, and more efficient energy management, ultimately enhancing the overall reliability and resilience of the system.
Cost Savings and Improved Profitability
The enhanced efficiency, predictive capabilities, and automated decision-making enabled by AI can result in significant cost savings for renewable energy projects. By optimizing energy generation, reducing waste, and improving overall system performance, AI-powered solutions can contribute to improved profitability and a more favorable return on investment for renewable energy investors and operators.
Increased Integration and Grid Optimization
AI-powered renewable energy systems can also facilitate better integration with existing power grids, enabling more efficient load balancing, energy storage optimization, and grid-scale management. This can lead to improved grid stability, reduced energy losses, and a more seamless transition towards a decarbonized energy system.
Challenges of Implementing AI in Renewable Energy
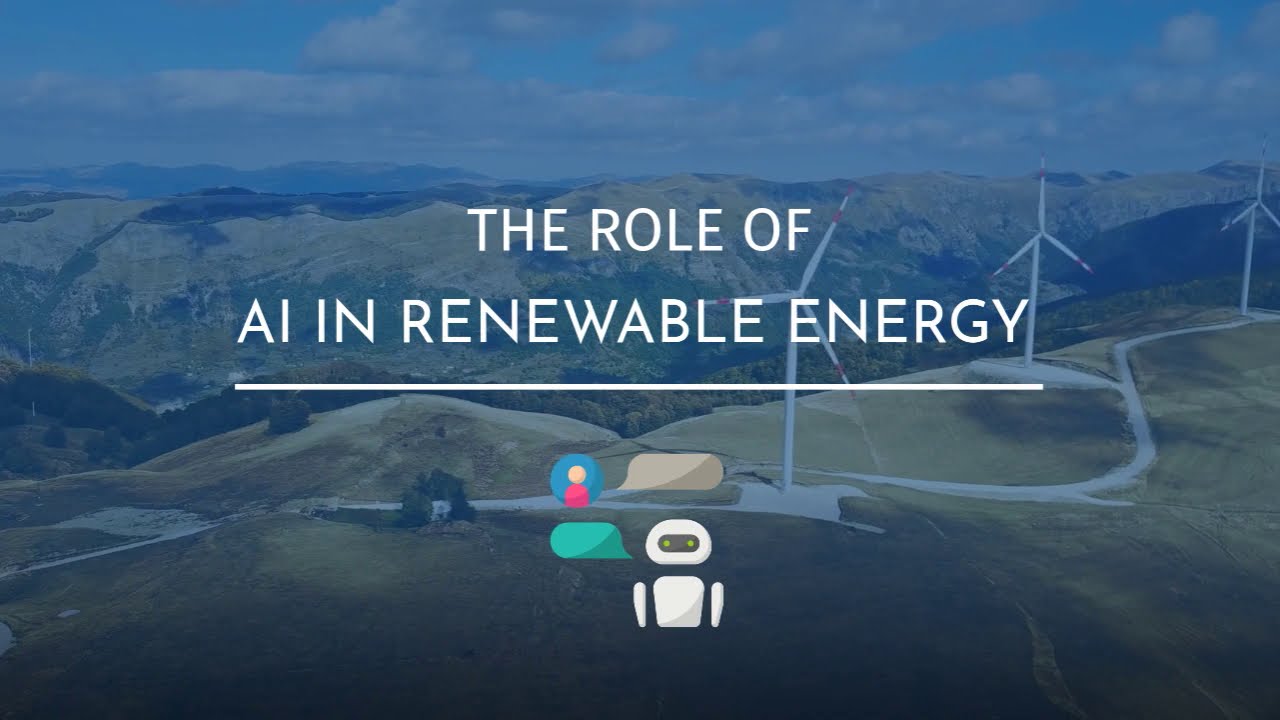
Data Availability and Quality
The effective implementation of AI in renewable energy relies heavily on the availability and quality of data. Acquiring, aggregating, and maintaining high-quality datasets from various sources can be a significant challenge, particularly in regions with limited sensor infrastructure or historical data.
Technological Complexity and Integration
Integrating AI-powered solutions into existing renewable energy systems can be a complex and time-consuming process. Ensuring smooth integration, seamless data exchange, and the effective deployment of AI algorithms can require significant technical expertise and coordination between various stakeholders.
Regulatory and Policy Considerations
The adoption of AI in renewable energy may also be influenced by regulatory and policy frameworks. Navigating the evolving landscape of data privacy, cybersecurity, and energy market regulations can pose challenges for renewable energy operators and AI solution providers.
Talent and Skill Gaps
The successful implementation of AI in renewable energy requires a specialized workforce with expertise in both renewable energy technologies and AI/machine learning. Addressing the talent and skill gaps in this emerging field can be a significant hurdle for organizations seeking to leverage AI-powered solutions.
Scalability and Deployment Challenges
As the demand for AI-powered renewable energy solutions grows, scalability and efficient deployment become critical factors. Ensuring that AI-based systems can be scaled up to handle increasing volumes of data and energy generation while maintaining performance and reliability is an ongoing challenge.
Case Studies of AI Optimization in Renewable Energy
Solar Energy Optimization
Predictive Maintenance and Asset Management
A leading solar energy company has implemented an AI-powered predictive maintenance system to monitor the performance of its photovoltaic panels. By analyzing sensor data, weather forecasts, and historical maintenance records, the system can identify potential issues before they occur, allowing for proactive maintenance and improved asset longevity.
Automated Shading Optimization
Another solar energy provider has deployed an AI-powered system that dynamically adjusts the orientation and tilt of solar panels based on real-time shading conditions and energy demand. This optimization has resulted in a significant increase in energy generation, with a corresponding boost in profitability.
Wind Energy Optimization
Wind Turbine Performance Optimization
A major wind farm operator has leveraged AI algorithms to optimize the individual performance of wind turbines within their grid. By analyzing sensor data, weather patterns, and operational parameters, the system can dynamically adjust blade pitch, rotor speed, and other variables to maximize energy output and minimize maintenance costs.
Predictive Maintenance and Failure Prevention
In the wind energy sector, AI is also being used to predict and prevent equipment failures. By monitoring vibration patterns, component wear, and environmental factors, AI-powered systems can identify potential issues before they lead to costly downtime or equipment replacement.
Hydroelectric Power Optimization
Reservoir Level Management
A hydroelectric power plant has implemented an AI-powered system to optimize its reservoir level management. By combining historical data, weather forecasts, and real-time sensor inputs, the system can predict water flow, anticipate demand, and make automated adjustments to water release and storage, ensuring efficient energy generation while maintaining sustainability.
Turbine Efficiency Optimization
Another hydroelectric plant has leveraged AI to enhance the efficiency of its turbines. By continuously monitoring parameters such as water flow, pressure, and vibration, the AI system can fine-tune turbine operations, leading to increased energy output and reduced maintenance requirements.
Future Trends in AI for Renewable Energy
Advancements in Deep Learning and Neural Networks
As AI technology continues to evolve, the integration of advanced techniques like deep learning and neural networks is expected to drive further improvements in renewable energy optimization. These algorithms can uncover increasingly complex patterns and relationships within large, diverse datasets, enabling even more precise and adaptive energy management solutions.
Integration with IoT and Edge Computing
The convergence of AI, the Internet of Things (IoT), and edge computing is poised to transform the renewable energy landscape. By leveraging IoT-enabled sensors and edge computing capabilities, AI-powered systems can process data closer to the source, enabling real-time decision-making and rapid response to changing conditions.
Hybrid AI-Human Decision-Making
While AI will continue to play a dominant role in renewable energy optimization, the future is likely to see a greater emphasis on hybrid AI-human decision-making. By combining the computational power and analytical capabilities of AI with the contextual understanding and strategic decision-making of human experts, renewable energy systems can achieve an even higher level of efficiency and resilience.
Integrated Energy Storage and Grid Management
As the integration of renewable energy sources into the grid increases, AI-powered solutions will play a crucial role in managing energy storage systems and optimizing grid-scale operations. AI algorithms can help balance supply and demand, coordinate energy storage and distribution, and ensure the overall stability and reliability of the energy network.
Sustainable AI Development
As the adoption of AI in renewable energy grows, there will be an increased focus on developing AI systems that align with sustainability goals. This includes exploring energy-efficient AI hardware, optimizing AI algorithms for reduced carbon footprints, and ensuring the responsible and ethical use of AI in the renewable energy sector.
Conclusion
The integration of AI and renewable energy represents a promising path towards a more sustainable and efficient energy future. By leveraging the power of AI-driven optimization, prediction, and decision-making, renewable energy systems can achieve unprecedented levels of performance, reliability, and cost-effectiveness.
As the industry continues to evolve, the successful implementation of AI in renewable energy will require overcoming challenges related to data availability, technological integration, regulatory frameworks, and talent development. However, the potential benefits of AI-powered renewable energy solutions are vast, and the future holds exciting possibilities for the seamless integration of these transformative technologies.