The rapid growth of social media and user-generated content has led to an unprecedented amount of data available on consumer opinions, sentiments, and preferences. This has paved the way for the development of advanced techniques in sentiment analysis and opinion mining, with Artificial Intelligence (AI) playing a crucial role in these fields.
Overview of AI in Sentiment Analysis
AI-Powered Sentiment Analysis
Sentiment analysis is the process of identifying and extracting subjective information, such as opinions, attitudes, and emotions, from text data. AI has significantly enhanced the capabilities of sentiment analysis by providing more accurate and nuanced approaches to understanding the underlying sentiment in various forms of textual content.
Machine Learning in Sentiment Analysis
Machine learning algorithms, such as Support Vector Machines (SVM), Naive Bayes, and Recurrent Neural Networks (RNNs), have been widely adopted in sentiment analysis. These algorithms are trained on large datasets of labeled text data, enabling them to learn patterns and relationships between linguistic features and sentiment polarity (positive, negative, or neutral).
Natural Language Processing (NLP) in Sentiment Analysis
NLP techniques, such as tokenization, part-of-speech tagging, and sentiment lexicon analysis, are crucial in sentiment analysis. AI-powered NLP models can analyze the syntactic and semantic structure of text, allowing for a more comprehensive understanding of the underlying sentiment.
Deep Learning in Sentiment Analysis
The advancements in deep learning have further improved the performance of sentiment analysis. Deep learning models, such as Convolutional Neural Networks (CNNs) and Long Short-Term Memory (LSTMs), can capture complex patterns and nuances in language, leading to more accurate sentiment predictions.
Multimodal Sentiment Analysis
AI-driven sentiment analysis is not limited to textual data; it can also be applied to various other data modalities, such as images, videos, and audio. Multimodal sentiment analysis combines different data sources to gain a more comprehensive understanding of user sentiment.
Applications of AI-Powered Sentiment Analysis
AI-powered sentiment analysis has a wide range of applications across various industries, including:
- Customer Experience Management: Analyzing customer feedback, reviews, and social media interactions to understand customer sentiment and improve product and service offerings.
- Brand Reputation Monitoring: Tracking and analyzing mentions of a brand or product on social media and online platforms to manage brand reputation and identify potential crisis situations.
- Market Research and Trend Analysis: Extracting insights from customer opinions and preferences to inform business strategy, product development, and marketing campaigns.
- Political and Social Discourse Analysis: Analyzing public sentiment towards political leaders, policies, and social issues to understand public opinion and inform decision-making.
- Personalized Recommendation Systems: Utilizing sentiment analysis to provide tailored product or content recommendations based on user preferences and opinions.
Importance of Opinion Mining
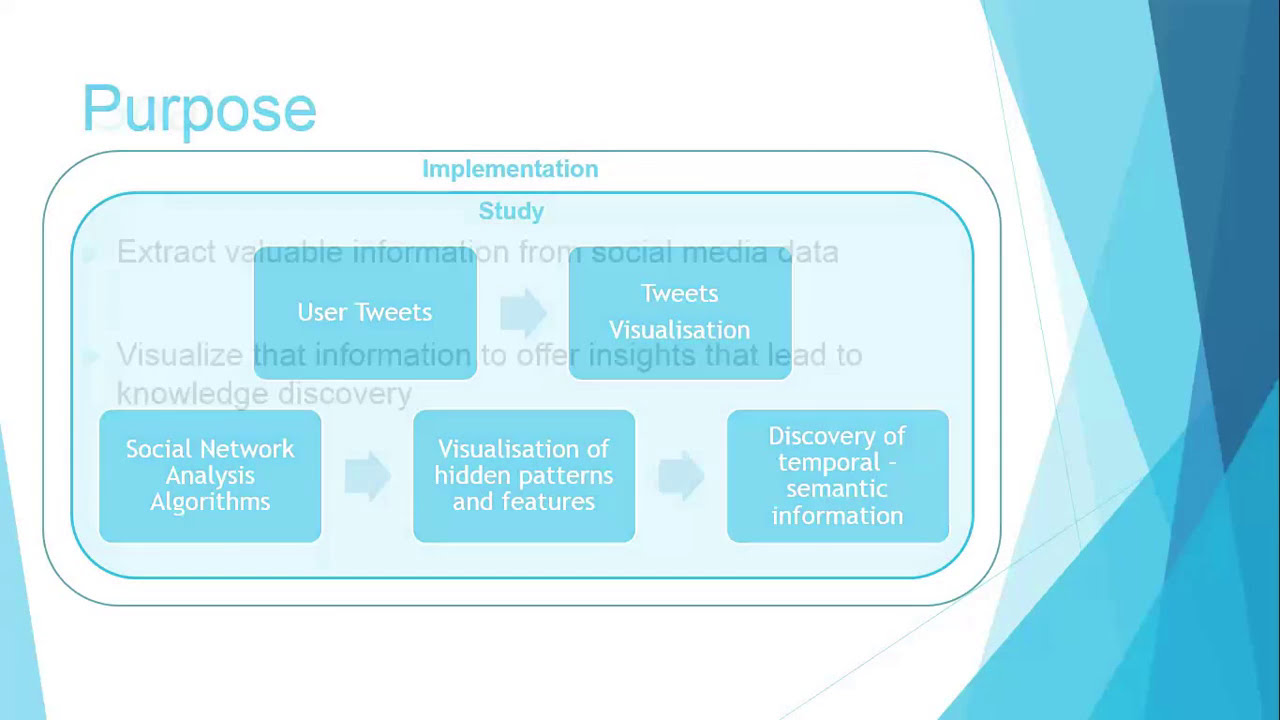
Understanding Consumer Behavior
Opinion mining provides valuable insights into consumer behavior, preferences, and decision-making processes. By analyzing the sentiments and opinions expressed by consumers, businesses can gain a deeper understanding of their target audience, anticipate market trends, and make more informed strategic decisions.
Informed Decision-Making
Opinion mining enables organizations to make data-driven decisions by leveraging the insights extracted from vast amounts of user-generated content. This can lead to improved product development, better customer service, and more effective marketing and advertising strategies.
Reputation Management
In today’s digital landscape, where information and opinions spread rapidly, opinion mining plays a crucial role in monitoring and managing the reputation of brands, products, and organizations. By tracking and analyzing sentiment towards a particular entity, businesses can proactively address any negative sentiment and maintain a positive brand image.
Competitive Intelligence
Opinion mining can provide valuable competitive intelligence by revealing insights into consumer perceptions of competing products or services. This information can be used to identify areas for improvement, differentiate offerings, and develop more effective strategies to gain a competitive edge.
Personalized Experiences
By understanding individual preferences and sentiments, opinion mining can enable the creation of personalized experiences for customers. This can lead to increased customer satisfaction, loyalty, and engagement, ultimately driving business growth.
Applications of AI in Sentiment Analysis and Opinion Mining
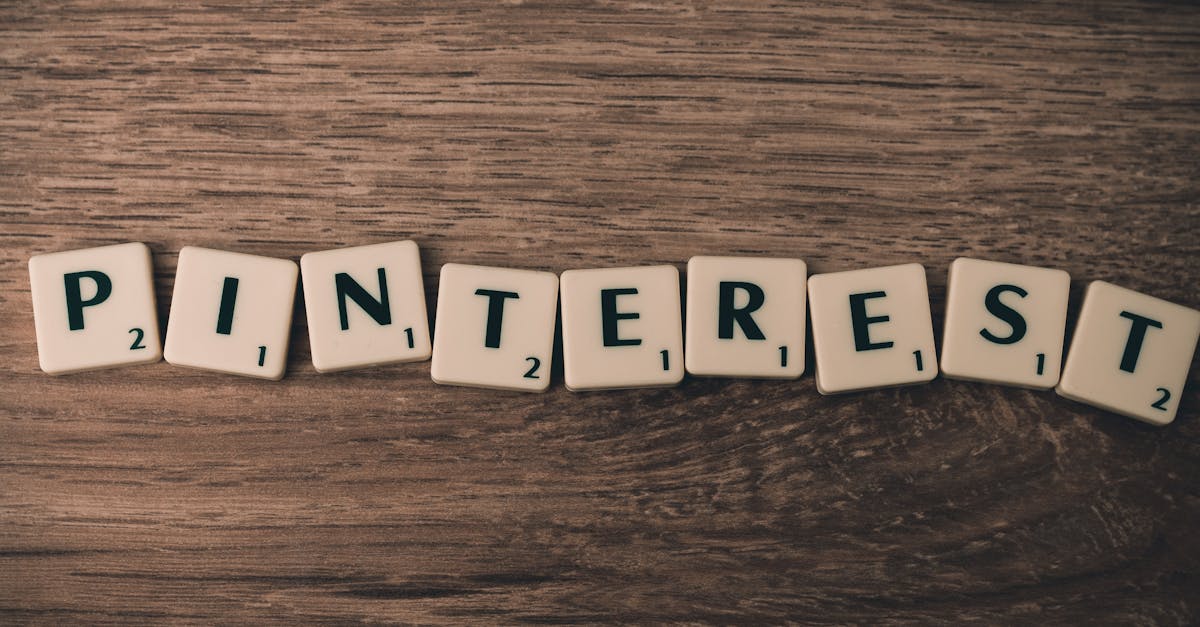
Customer Experience Management
Customer Feedback Analysis
AI-powered sentiment analysis can be used to analyze customer feedback, reviews, and complaints across various channels, such as customer service interactions, social media, and online platforms. This can help organizations identify pain points, address customer concerns, and improve overall customer satisfaction.
Proactive Issue Identification
AI can detect and flag emerging issues or negative sentiment trends in real-time, enabling organizations to respond quickly and address customer concerns before they escalate.
Personalized Recommendations
Sentiment analysis can be combined with other customer data to provide personalized product or service recommendations, enhancing the customer experience and increasing customer loyalty.
Brand Reputation Monitoring
Social Media Monitoring
AI-driven sentiment analysis can be used to monitor brand mentions, customer conversations, and influencer activities on social media platforms. This can help organizations quickly identify and address any potential reputational risks or negative sentiment.
Online Reputation Management
By analyzing online reviews, news articles, and forum discussions, AI can provide insights into the overall perception of a brand or product, enabling organizations to develop targeted strategies to enhance their online reputation.
Crisis Management
AI-powered sentiment analysis can help organizations detect and respond to potential crisis situations in a timely manner, mitigating the impact on brand reputation and public perception.
Market Research and Trend Analysis
Competitor Benchmarking
AI can be used to analyze the sentiment and opinions expressed towards competing products or services, enabling organizations to identify areas for differentiation and improve their competitive positioning.
Product Development Insights
Sentiment analysis of customer feedback can provide valuable insights into product features, pain points, and areas for improvement, informing the product development process and ensuring the creation of products that better meet customer needs.
Trend Identification
AI-driven sentiment analysis can help organizations detect emerging trends, consumer preferences, and shifts in market sentiment, allowing them to adapt their strategies and stay ahead of the competition.
Political and Social Discourse Analysis
Public Opinion Tracking
AI-powered sentiment analysis can be used to monitor and analyze public sentiment towards political leaders, policies, and social issues, enabling a better understanding of the underlying sentiments and concerns of the electorate.
Misinformation and Fake News Detection
Sentiment analysis combined with other AI techniques, such as natural language processing and machine learning, can help identify and mitigate the spread of misinformation and fake news on social media and online platforms.
Targeted Messaging and Outreach
By understanding the sentiment and opinions of different demographic groups, organizations and political entities can develop more effective and targeted messaging and outreach strategies.
Challenges in AI Sentiment Analysis and Opinion Mining
Data Quality and Diversity
Accurate sentiment analysis and opinion mining rely on the quality and diversity of the data used to train the AI models. Challenges include handling noisy or biased data, addressing language and cultural differences, and dealing with the evolving nature of language and sentiment.
Contextual Understanding
Sentiment analysis requires a deep understanding of the context in which language is used, as the same words or phrases can convey different meanings and sentiments in different contexts. Developing AI models that can accurately capture and interpret contextual nuances is an ongoing challenge.
Multimodal Sentiment Analysis
Extending sentiment analysis beyond textual data to include other modalities, such as images, videos, and audio, introduces additional challenges in data integration, feature extraction, and model development.
Ethical Considerations
As sentiment analysis and opinion mining become more prevalent, there are growing concerns about the ethical use of such technologies, including privacy, data bias, and the potential for misuse or manipulation of public opinion.
Scalability and Real-Time Processing
With the ever-increasing volume of user-generated content, sentiment analysis and opinion mining systems need to be scalable and capable of processing data in real-time to provide timely and relevant insights.
Interpretability and Explainability
While AI-powered sentiment analysis can provide valuable insights, there is a growing need for greater interpretability and explainability of the underlying models and decision-making processes to ensure transparency and trust in the results.
Future Trends in the Field
Advancements in Deep Learning
Continued advancements in deep learning, including the development of more sophisticated neural network architectures and the availability of larger, more diverse datasets, are expected to lead to significant improvements in the accuracy and robustness of sentiment analysis and opinion mining models.
Multimodal Sentiment Analysis
The integration of different data modalities, such as text, images, audio, and video, will become increasingly important in sentiment analysis and opinion mining, enabling a more comprehensive understanding of user sentiment and behavior.
Personalization and Contextual Understanding
AI-powered sentiment analysis will continue to evolve towards more personalized and context-aware systems, leveraging user profiles, behavioral data, and other contextual information to provide more accurate and relevant insights.
Ethical and Responsible AI
As the use of sentiment analysis and opinion mining becomes more widespread, there will be a growing emphasis on developing ethical and responsible AI practices, ensuring the transparent and accountable use of these technologies while addressing concerns around privacy, bias, and the potential for misuse.
Hybrid Approaches
Combining traditional rule-based sentiment analysis techniques with advanced AI-powered approaches, such as machine learning and deep learning, will lead to the development of hybrid systems that can provide more accurate and reliable sentiment analysis and opinion mining.
Real-Time Monitoring and Predictive Capabilities
Advancements in computational power and data processing capabilities will enable sentiment analysis and opinion mining systems to operate in real-time, providing faster insights and allowing organizations to respond more quickly to changing market conditions and consumer sentiment.
Conclusion
AI-powered sentiment analysis and opinion mining have become increasingly crucial in today’s data-driven world, where understanding consumer sentiment and preferences is essential for business success. By leveraging the capabilities of AI, organizations can gain valuable insights into customer behavior, monitor brand reputation, inform strategic decision-making, and deliver personalized experiences.
As the field continues to evolve, the integration of advanced deep learning techniques, multimodal data sources, and contextual understanding will drive further advancements in sentiment analysis and opinion mining. At the same time, the ethical and responsible development of these technologies will be of paramount importance, ensuring that they are used in a transparent and accountable manner to benefit both businesses and consumers.