Advances in technology have revolutionized the healthcare industry, and the field of mental health is no exception. The integration of Artificial Intelligence (AI) into mental health diagnosis has the potential to transform the way we approach and manage psychological well-being. This article will explore the role of AI in mental health diagnosis, the benefits and challenges of its implementation, and the future implications of this emerging technology.
Overview of AI in Healthcare
Artificial Intelligence is a rapidly evolving field that has found numerous applications in the healthcare industry. From drug discovery to medical imaging analysis, AI has demonstrated its ability to enhance diagnostic accuracy, streamline workflows, and improve patient outcomes. In the realm of mental health, AI-powered tools are being developed to assist clinicians in the assessment, diagnosis, and treatment of various psychological conditions.
AI in Medical Diagnosis
AI algorithms, such as machine learning and deep learning, have shown promising results in analyzing large datasets, identifying patterns, and making accurate predictions. In the medical field, these technologies have been used to analyze medical images, patient records, and other clinical data to aid in the diagnosis of various diseases, including cancer, cardiovascular disorders, and neurological conditions.
Benefits of AI in Healthcare
The integration of AI in healthcare has brought about several benefits, including:
- Improved diagnostic accuracy
- Faster decision-making
- Reduced workload for healthcare professionals
- Personalized and targeted treatments
- Increased accessibility to healthcare services
Importance of Mental Health Diagnosis

Mental health disorders are a significant public health concern, affecting millions of individuals worldwide. Accurate and timely diagnosis is crucial for providing appropriate treatment and support, as well as for understanding the prevalence and impact of these conditions.
Prevalence and Impact of Mental Health Disorders
Mental health disorders, such as depression, anxiety, bipolar disorder, and schizophrenia, are widespread and can have a profound impact on an individual’s quality of life, relationships, and overall well-being. According to the World Health Organization, mental health disorders are a leading cause of disability globally, and the COVID-19 pandemic has further exacerbated the mental health crisis.
Challenges in Mental Health Diagnosis
Diagnosing mental health disorders can be a complex and subjective process, often relying on self-reported symptoms, clinical assessments, and the expertise of mental health professionals. Factors such as stigma, access to healthcare, and the subjective nature of psychological evaluation can contribute to the challenges in accurate and timely diagnosis.
Current Methods of Mental Health Diagnosis
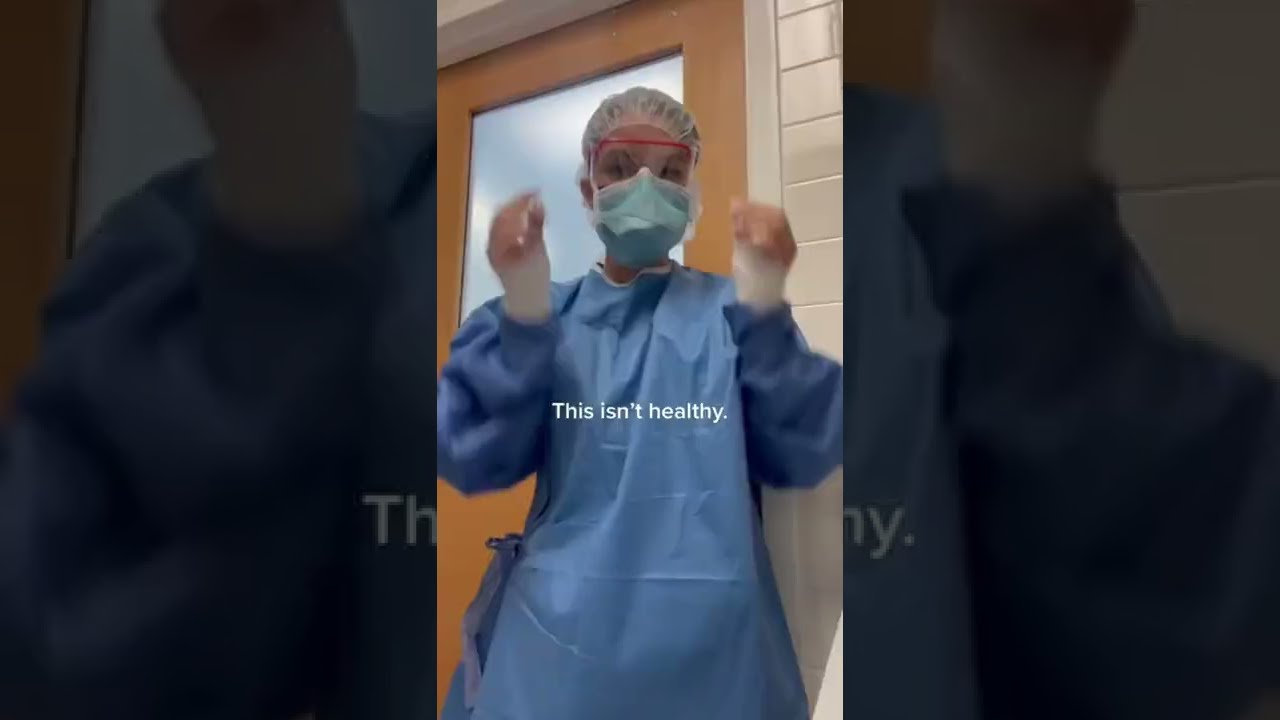
Traditionally, mental health professionals have relied on a combination of clinical interviews, psychological assessments, and diagnostic manuals, such as the Diagnostic and Statistical Manual of Mental Disorders (DSM) or the International Classification of Diseases (ICD), to diagnose mental health conditions.
Clinical Interviews and Assessments
Clinicians often conduct in-depth interviews with patients, gathering information about their symptoms, medical history, and life experiences. They may also administer various psychological tests and assessments to measure cognitive, emotional, and behavioral patterns.
Diagnostic Manuals and Criteria
The DSM and ICD provide standardized criteria and guidelines for the diagnosis of mental health disorders. These manuals outline the specific symptoms, duration, and impairment required for a particular diagnosis, helping to ensure consistency and reliability in the diagnostic process.
Limitations of Current Diagnostic Methods
While the existing methods of mental health diagnosis have been valuable, they also have their limitations. These include:
- Subjective nature of clinical assessments
- Reliance on self-reported symptoms
- Potential for inconsistency in diagnosis across clinicians
- Limited accessibility and availability of mental health services
Benefits of Using AI in Mental Health Diagnosis
The integration of AI in the field of mental health diagnosis offers several potential benefits, including:
Improved Diagnostic Accuracy
AI-powered algorithms can analyze vast amounts of data, including patient records, diagnostic tests, and other clinical information, to identify patterns and markers of mental health conditions. This can lead to more accurate and consistent diagnoses, reducing the risk of misdiagnosis or delayed diagnosis.
Faster and More Efficient Assessments
AI-based tools can automate certain aspects of the diagnostic process, such as administering and scoring psychological assessments, allowing clinicians to spend more time on therapeutic interventions and patient care.
Personalized and Targeted Treatments
By analyzing an individual’s unique data, AI can help identify specific treatment approaches that are best suited to their needs, leading to more personalized and effective interventions.
Increased Accessibility to Mental Health Services
AI-based tools can be integrated into mobile applications, telemedicine platforms, and other digital healthcare solutions, making mental health services more accessible to individuals who may have limited access to traditional in-person care.
Early Intervention and Prevention
AI algorithms can be trained to detect early signs and risk factors for mental health disorders, enabling earlier intervention and potentially preventing the development of more severe conditions.
Challenges and Limitations of AI in Mental Health Diagnosis
While the potential benefits of AI in mental health diagnosis are significant, there are also important challenges and limitations to consider:
Ethical Considerations
The use of AI in healthcare raises ethical concerns, such as issues of privacy, data security, and the potential for bias and discrimination. Mental health data is particularly sensitive, and it is crucial to ensure that AI systems are designed and deployed in a way that protects patient privacy and respects ethical principles.
Interpretability and Explainability
Many AI models, particularly those based on deep learning, can be complex and difficult to interpret, making it challenging for clinicians to understand the reasoning behind the AI’s diagnostic recommendations. Developing more transparent and explainable AI systems is a crucial step in building trust and facilitating collaboration between AI and healthcare professionals.
Data Availability and Quality
The effectiveness of AI-based mental health diagnosis relies on the availability of high-quality, diverse, and representative datasets. Ensuring the diversity and accuracy of these datasets is essential to prevent biases and ensure the generalizability of the AI models.
Integration with Clinical Workflows
Seamlessly integrating AI-based tools into existing clinical workflows and ensuring their compatibility with electronic health record systems is a significant challenge that must be addressed to facilitate the adoption of these technologies in healthcare settings.
Regulatory Considerations
The use of AI in healthcare is subject to various regulatory guidelines and approval processes, which can vary across different jurisdictions. Navigating these regulatory frameworks and ensuring that AI-based diagnostic tools meet the necessary safety and efficacy standards is crucial for their successful implementation.
Case Studies and Examples
To illustrate the potential of AI in mental health diagnosis, let’s explore a few case studies and examples:
Depression Screening Using Voice Analysis
Researchers have developed AI-based systems that can analyze an individual’s voice patterns to detect signs of depression. These systems leverage machine learning algorithms to identify subtle changes in vocal characteristics, such as pitch, tone, and speech patterns, that may be indicative of depressive symptoms.
Anxiety Disorder Diagnosis Using Facial Expression Analysis
Another example is the use of AI to analyze facial expressions and micro-expressions to assist in the diagnosis of anxiety disorders. By training AI models on large datasets of facial expressions, researchers have been able to develop tools that can detect subtle changes in facial features that may be associated with different types of anxiety disorders.
Early Detection of Psychosis Using Natural Language Processing
AI-powered natural language processing (NLP) has been explored for the early detection of psychotic disorders, such as schizophrenia. By analyzing the language patterns and semantic content of an individual’s speech or written text, these AI systems can identify subtle changes that may be indicative of the onset of psychosis, enabling earlier intervention and potentially better outcomes.
Personalized Treatment Recommendations Using Multimodal Data
Combining multiple data sources, such as clinical assessments, genetic information, and neuroimaging data, AI algorithms can be trained to provide personalized treatment recommendations for mental health conditions. This approach aims to match individuals with the most appropriate therapies, medications, or other interventions based on their unique characteristics and response patterns.
Future Implications and Possibilities
As the field of AI in mental health diagnosis continues to evolve, the potential future implications and possibilities are vast. Here are some areas to consider:
Integrating AI into Clinical Decision-Making
The seamless integration of AI-based tools into the clinical decision-making process will be a crucial step in the widespread adoption of these technologies. Developing robust and trustworthy AI systems that can collaborate with healthcare professionals, rather than replace them, will be essential.
Personalized and Adaptive Mental Health Interventions
With the ability to analyze comprehensive data about an individual’s mental health, AI-powered systems can pave the way for highly personalized and adaptive interventions. This could involve tailoring treatments, medication dosages, and therapeutic approaches to an individual’s unique needs and responses.
Continuous Monitoring and Early Intervention
AI-enabled wearable devices and mobile applications can provide continuous monitoring of an individual’s mental health, allowing for early detection of emerging issues and timely intervention. This could lead to improved long-term outcomes and prevention of more severe mental health conditions.
Enhancing Mental Health Research and Drug Discovery
The use of AI in mental health research can accelerate the discovery of new therapeutic targets, the identification of biomarkers, and the development of more effective medications and treatments. By analyzing large datasets and identifying complex patterns, AI can aid in the design of more efficient and targeted clinical trials.
Addressing Mental Health Disparities
AI-based tools have the potential to improve access to mental health services, particularly for underserved or marginalized communities. By overcoming barriers such as geographical location, language, and socioeconomic status, AI-powered solutions can help bridge the gap in mental healthcare delivery.
Conclusion
The integration of Artificial Intelligence in mental health diagnosis holds immense promise for transforming the way we approach and manage psychological well-being. By leveraging the power of AI to enhance diagnostic accuracy, streamline assessment processes, and personalize treatment approaches, we can unlock new possibilities for improving mental health outcomes and addressing the significant challenges faced in this field.
However, the successful implementation of AI in mental health diagnosis requires careful consideration of the ethical, technical, and regulatory challenges. Ensuring the transparency, fairness, and reliability of these AI-powered systems, while maintaining the trust and collaboration of healthcare professionals, is essential. As the field continues to evolve, the future implications of AI in mental health diagnosis extend beyond improved clinical outcomes, potentially leading to advancements in research, drug discovery, and the reduction of mental health disparities.
As we navigate this exciting and rapidly evolving landscape, it is crucial that we approach the integration of AI in mental health diagnosis with a balanced and thoughtful approach, prioritizing the well-being and privacy of individuals, while harnessing the transformative potential of this technology to revolutionize the way we understand, diagnose, and treat mental health conditions.