Artificial Intelligence (AI) has been making significant strides in the field of healthcare, and one area that has seen particularly impressive advancements is neurology. The intersection of AI and neurology holds immense promise, as the ability to analyze and interpret complex neurological data can lead to more accurate diagnoses, personalized treatment plans, and improved patient outcomes.
Introduction to AI in Neurology
Understanding the Potential of AI in Neurology
Neurology is a complex field that deals with the diagnosis and treatment of disorders affecting the brain, spinal cord, and nervous system. With the ever-increasing amount of data generated through advanced imaging techniques, genetic analysis, and electronic medical records, the need for efficient and accurate data processing has become more crucial than ever. This is where AI can play a transformative role.
The Convergence of AI and Neurology
AI algorithms, particularly in the areas of machine learning and deep learning, have shown remarkable capabilities in pattern recognition, image analysis, and data processing. These techniques can be applied to neurological data, such as MRI scans, EEG signals, and genomic information, to identify subtle patterns and insights that may be overlooked by human experts.
The Potential for AI-Driven Breakthroughs
By harnessing the power of AI, neurologists can gain a deeper understanding of the underlying mechanisms of neurological disorders, leading to more accurate diagnoses, personalized treatment approaches, and improved patient outcomes. This convergence of AI and neurology holds the potential to revolutionize the way we approach neurological care.
Applications of AI in Neurology
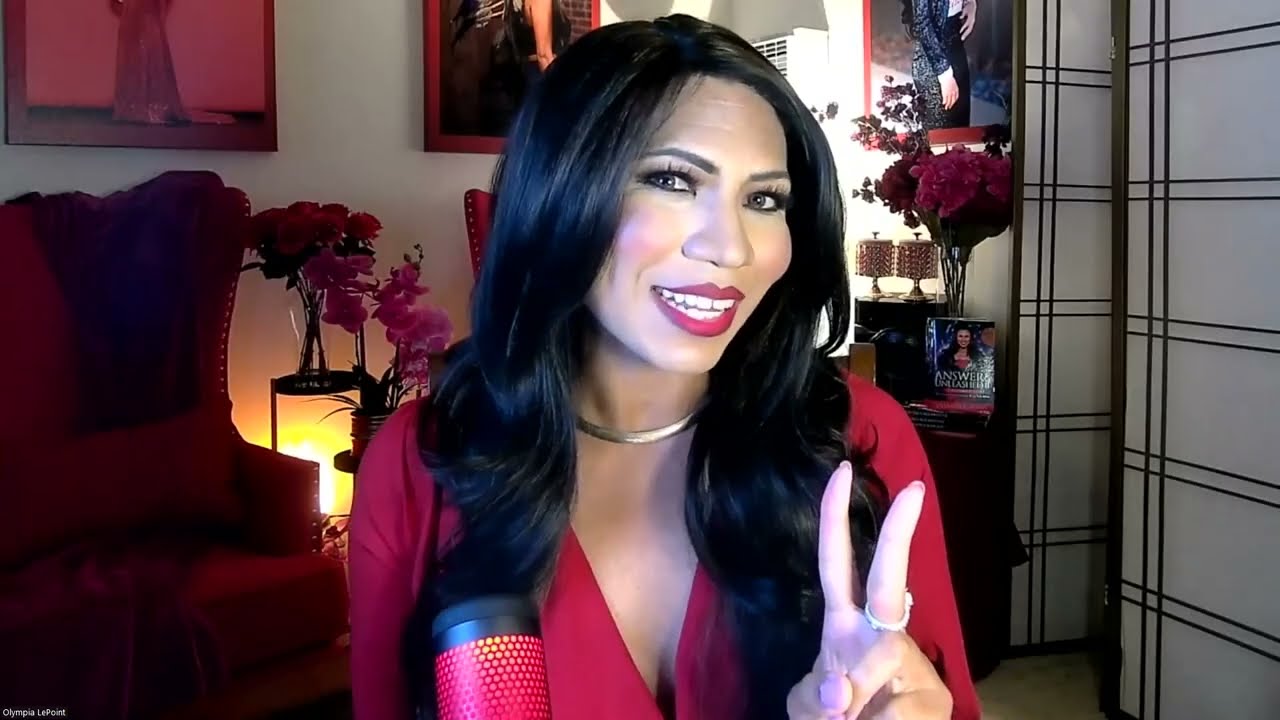
Neuroimaging and Diagnostics
One of the most prominent applications of AI in neurology is in the field of neuroimaging. AI algorithms can be trained to analyze medical images, such as MRI, CT, and PET scans, with greater accuracy and speed than human experts. This can lead to earlier and more precise diagnoses of conditions like Alzheimer’s disease, Parkinson’s disease, brain tumors, and stroke.
Improved Lesion Detection
AI-powered image analysis can help identify and characterize lesions in the brain, spinal cord, and nervous system with greater precision. This can lead to earlier detection of neurological disorders, enabling timely interventions and improved patient outcomes.
Condition | Description | AI Application |
---|---|---|
Alzheimer’s Disease | Neurodegenerative disorder affecting memory and cognitive function | AI can detect changes in brain structure and function, aiding early diagnosis |
Parkinson’s Disease | Movement disorder characterized by tremors, stiffness, and impaired motor function | AI can analyze patterns in brain imaging data to assist with diagnosis |
Brain Tumors | Abnormal growth of cells in the brain, which can be benign or malignant | AI can identify and characterize brain tumors, guiding treatment decisions |
Automated Segmentation and Quantification
AI algorithms can be trained to segment and quantify specific brain structures and lesions, providing valuable information to neurologists for diagnosis, treatment planning, and monitoring disease progression.
- Automated segmentation of brain regions
- Quantification of disease-specific biomarkers
- Longitudinal tracking of structural changes
Neurophysiological Data Analysis
In addition to neuroimaging, AI can also be applied to the analysis of neurophysiological data, such as electroencephalography (EEG) and electromyography (EMG). These techniques can provide insights into the electrical activity of the brain and neuromuscular system, respectively.
Detection of Abnormal Brain Activity
AI-powered EEG analysis can help identify patterns of abnormal brain activity associated with various neurological disorders, including epilepsy, sleep disorders, and neurodegenerative diseases.
Prediction of Seizures and Other Events
By analyzing EEG data, AI algorithms can potentially predict the onset of seizures, allowing for timely intervention and improved management of epilepsy.
Personalized Rehabilitation Strategies
AI-driven EMG analysis can aid in the development of personalized rehabilitation strategies for patients with neuromuscular disorders, such as stroke, spinal cord injury, and Parkinson’s disease.
Genomics and Precision Medicine
The field of neurogenomics, which combines neurology and genomics, has also benefited from the integration of AI. By analyzing genetic data, AI algorithms can help identify genetic markers associated with neurological disorders, enabling more accurate diagnoses and the development of personalized treatment plans.
Genetic Risk Prediction
AI models can analyze an individual’s genetic profile to assess their risk of developing certain neurological disorders, such as Alzheimer’s disease or Parkinson’s disease, allowing for early intervention and preventive measures.
Pharmacogenomics
AI can be used to integrate genetic information with drug response data, enabling the optimization of medication dosages and the selection of the most effective treatments for individual patients, reducing the risk of adverse effects.
Biomarker Discovery
AI-driven analysis of genetic and molecular data can lead to the identification of novel biomarkers for neurological disorders, which can inform diagnosis, prognosis, and the development of new therapeutic targets.
Benefits of AI in Neurology
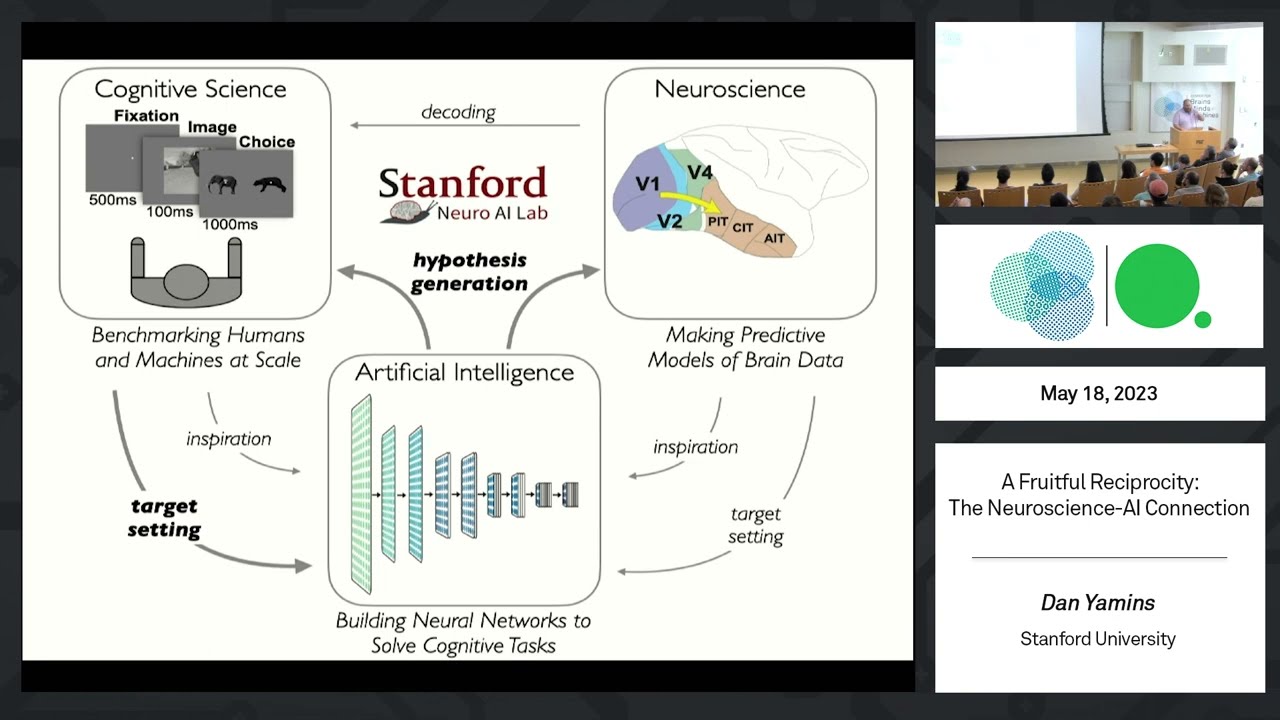
Improved Diagnostic Accuracy
One of the primary benefits of AI in neurology is its ability to improve diagnostic accuracy. By leveraging advanced algorithms and vast amounts of data, AI can detect subtle patterns and identify markers that may be missed by human experts, leading to earlier and more precise diagnoses.
Enhanced Personalized Treatment
AI can also play a significant role in developing personalized treatment plans for neurological patients. By analyzing individual genetic profiles, neuroimaging data, and other relevant information, AI can help select the most appropriate therapies, optimize drug dosages, and predict treatment outcomes.
Accelerated Research and Drug Discovery
AI-powered tools can expedite the process of neurological research and drug discovery. By analyzing large datasets, AI algorithms can identify potential therapeutic targets, screen drug candidates, and predict the efficacy and safety of new treatments.
Improved Patient Outcomes
Ultimately, the integration of AI in neurology can lead to improved patient outcomes. By enabling earlier diagnosis, personalized treatment, and more efficient research, AI can contribute to better management of neurological disorders, reduced patient suffering, and enhanced quality of life.
Challenges and Limitations of AI in Neurology
Data Availability and Quality
The successful application of AI in neurology relies on the availability of high-quality, comprehensive datasets. Addressing issues related to data availability, curation, and standardization is crucial for ensuring the reliability and generalizability of AI models.
Interpretability and Explainability
Many AI models, particularly those based on deep learning, are often viewed as “black boxes” due to their complex decision-making processes. Improving the interpretability and explainability of these models is essential for building trust and ensuring that neurologists can understand and validate the AI’s recommendations.
Regulatory and Ethical Considerations
The integration of AI in healthcare, including neurology, raises important regulatory and ethical concerns. Issues such as data privacy, bias, and the responsibility for AI-driven decisions must be carefully addressed to ensure the safe and ethical deployment of these technologies.
Integration with Clinical Workflows
Seamlessly integrating AI-powered tools into the existing clinical workflows of neurologists can be a significant challenge. Addressing technical, organizational, and cultural barriers is crucial for ensuring the successful adoption and effective utilization of AI in neurological practice.
Validation and Clinical Evidence
Robust validation and the demonstration of clinical evidence are essential for the widespread adoption of AI in neurology. Rigorous clinical studies and real-world evaluation are necessary to validate the performance and safety of AI-based tools and to demonstrate their tangible benefits in improving patient outcomes.
Future Implications of AI in Neurology
Advancements in Early Diagnosis and Preventive Care
As AI continues to evolve, its ability to detect neurological disorders at earlier stages will likely improve, enabling more timely interventions and potentially preventing the progression of these conditions.
Personalized Neurological Therapies
AI-driven personalization of neurological treatments, including drug selection, dosage optimization, and the development of novel therapies, will likely become more prevalent, leading to better patient outcomes and reduced adverse effects.
Automated Decision Support Systems
AI-powered decision support systems may become increasingly integrated into the daily practice of neurologists, providing real-time assistance in diagnosis, treatment planning, and patient management.
Enhanced Neurological Rehabilitation
AI can play a crucial role in the development of advanced rehabilitation technologies, such as brain-computer interfaces and robotic assistive devices, to improve the functional recovery of patients with neurological disorders.
Accelerated Neurological Research and Drug Discovery
The application of AI in neurological research and drug discovery is expected to continue to accelerate, leading to the identification of new targets, the development of more effective treatments, and the optimization of clinical trials.
Conclusion
The integration of Artificial Intelligence (AI) in the field of neurology has the potential to revolutionize the way neurological disorders are diagnosed, treated, and managed. By leveraging advanced algorithms and data-driven insights, AI can enhance diagnostic accuracy, enable personalized treatment approaches, accelerate research and drug discovery, and ultimately improve patient outcomes.
While the implementation of AI in neurology faces certain challenges and limitations, such as data availability, model interpretability, and regulatory concerns, the potential benefits outweigh the obstacles. As the technology continues to evolve and the evidence for its clinical utility grows, the future of AI in neurology looks increasingly promising.
The convergence of AI and neurology holds the promise of transforming the way we understand and address the complex conditions of the brain, spinal cord, and nervous system. By embracing this revolutionary technology, neurologists can unlock new avenues for research, enhance their clinical decision-making, and ultimately provide better care for their patients.