Financial forecasting is a crucial aspect of business management, as it allows organizations to anticipate and prepare for future financial conditions. With the advent of artificial intelligence (AI), the field of financial forecasting has undergone a significant transformation, offering new opportunities and challenges. In this comprehensive blog post, we will explore the role of AI in financial forecasting, examining its benefits, challenges, and future trends.
Overview of Financial Forecasting
Financial forecasting is the process of estimating the future financial performance of a company or an organization. This includes projecting revenues, expenses, cash flows, and other financial metrics. Accurate financial forecasting is essential for decision-making, strategic planning, and risk management.
Importance of Financial Forecasting
- Budgeting and Planning: Financial forecasts provide the foundation for creating realistic budgets and strategic plans.
- Risk Management: Forecasting helps identify potential risks and uncertainties, enabling organizations to develop contingency plans.
- Investment Decisions: Accurate financial forecasts inform investment decisions, helping investors assess the viability and potential returns of different opportunities.
- Performance Monitoring: Comparing actual financial results to forecasts allows organizations to track their progress and identify areas for improvement.
Traditional Approaches to Financial Forecasting
Historically, financial forecasting has relied on a variety of techniques, including:
- Time-series Analysis: Using historical data to identify patterns and trends that can be extrapolated into the future.
- Regression Analysis: Establishing relationships between financial variables and using them to predict future outcomes.
- Expert Judgment: Relying on the expertise and experience of financial analysts and subject-matter experts to make informed projections.
While these traditional approaches have their merits, they can be time-consuming, labor-intensive, and subject to human biases and errors.
What is AI?

Artificial intelligence (AI) is a broad term that encompasses the development of computer systems capable of performing tasks that typically require human intelligence, such as learning, problem-solving, and decision-making. AI systems can analyze vast amounts of data, identify patterns, and make predictions with a high degree of accuracy.
Components of AI
- Machine Learning: The ability of computer systems to learn and improve from experience without being explicitly programmed.
- Natural Language Processing (NLP): The ability of computer systems to understand, interpret, and generate human language.
- Computer Vision: The ability of computer systems to identify and process digital images and videos.
- Robotics: The development of autonomous or semi-autonomous machines capable of performing physical tasks.
Applications of AI
AI has a wide range of applications across various industries, including:
- Healthcare: Diagnostic assistance, drug discovery, and personalized treatment planning.
- Retail: Personalized recommendations, demand forecasting, and inventory optimization.
- Transportation: Autonomous vehicles, traffic management, and logistics optimization.
- Finance: Fraud detection, investment portfolio optimization, and financial forecasting.
Benefits of Using AI in Financial Forecasting
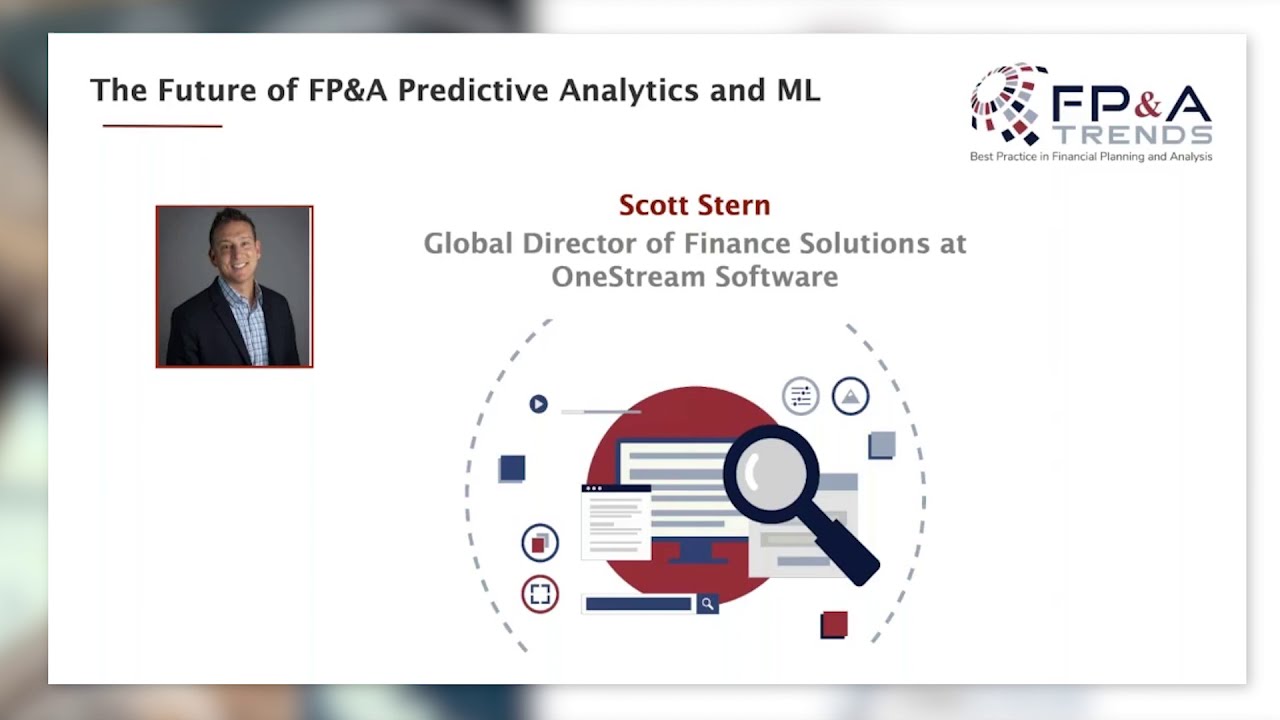
The integration of AI into financial forecasting has brought about several significant advantages:
Improved Accuracy
AI-powered financial forecasting models can analyze vast amounts of data, both structured and unstructured, to identify complex patterns and relationships that may be difficult for human analysts to detect. This can lead to more accurate and reliable forecasts.
Enhanced Speed and Efficiency
AI systems can process and analyze data much faster than human analysts, enabling organizations to make more timely and informed decisions. This can be particularly beneficial in fast-paced financial markets.
Reduced Bias and Subjectivity
AI-based forecasting models are less prone to the biases and subjective judgments that can influence human decision-making. This helps to ensure that forecasts are based on objective, data-driven insights.
Scenario Planning and Risk Assessment
AI can be used to simulate various scenarios and assess the potential impact of different variables on financial performance. This allows organizations to better understand and mitigate risks.
Personalized Insights
AI can tailor financial forecasts to the unique needs and characteristics of individual organizations, taking into account their specific market conditions, industry trends, and business strategies.
Continuous Learning and Adaptation
AI-based forecasting models can continuously learn from new data and adapt their algorithms, allowing them to improve their accuracy and relevance over time.
Challenges of Using AI in Financial Forecasting
While the benefits of using AI in financial forecasting are substantial, there are also several challenges and considerations to address:
Data Quality and Availability
The accuracy of AI-powered financial forecasts is heavily dependent on the quality and availability of the underlying data. Organizations must ensure that their data is clean, comprehensive, and up-to-date.
Transparency and Interpretability
AI models can be complex and opaque, making it difficult to understand the reasoning behind their predictions. This can pose challenges in terms of compliance, trust, and explainability.
Regulatory and Ethical Considerations
The use of AI in financial forecasting must adhere to relevant regulations and ethical standards, particularly in areas such as data privacy, algorithmic bias, and fiduciary responsibilities.
Integration with Existing Systems
Seamlessly integrating AI-powered forecasting tools with an organization’s existing financial systems and workflows can be a significant challenge, requiring careful planning and implementation.
Talent and Skill Gaps
Effectively implementing and leveraging AI in financial forecasting requires specialized skills and knowledge, which can be in short supply in many organizations.
Cybersecurity and Resilience
AI-based financial forecasting systems can be vulnerable to cyber threats, such as data breaches and malicious attacks, which can compromise the integrity and reliability of the forecasts.
Case Studies and Examples
To better understand the practical applications of AI in financial forecasting, let’s examine a few real-world case studies and examples:
Case Study 1: Predictive Maintenance in the Manufacturing Industry
A large manufacturing company implemented an AI-powered predictive maintenance system to forecast equipment failures and optimize its maintenance schedule. By analyzing sensor data, historical maintenance records, and other relevant information, the AI model was able to identify patterns and predict when specific pieces of equipment were likely to break down. This allowed the company to proactively schedule maintenance and avoid costly unplanned downtime, resulting in significant cost savings and improved operational efficiency.
Example 2: Forecasting Stock Market Trends
Several financial institutions and hedge funds have leveraged AI and machine learning to develop advanced models for forecasting stock market trends and making investment decisions. These AI-powered systems can analyze vast amounts of financial data, including market news, social media sentiment, and macroeconomic indicators, to identify patterns and make predictions about future stock price movements. While no forecasting model is 100% accurate, these AI-based approaches have demonstrated the potential to outperform traditional investment strategies.
Case Study 3: Personalized Wealth Management
A wealth management firm has implemented an AI-powered platform that provides personalized financial forecasts and investment recommendations to its clients. The AI system analyzes each client’s financial situation, risk tolerance, and investment goals, and then generates customized forecasts and portfolio optimization strategies. This has enabled the firm to offer a more tailored and efficient service to its clients, resulting in improved client satisfaction and increased asset under management.
Future Trends in AI-Driven Financial Forecasting
As AI technology continues to evolve, we can expect to see several exciting developments in the field of financial forecasting:
Increased Integration of AI and Big Data
The proliferation of big data, coupled with advancements in AI and machine learning, will enable more comprehensive and accurate financial forecasting models. Organizations will be able to leverage a wider range of data sources, including alternative data, to gain deeper insights and make more informed decisions.
Advancements in Natural Language Processing (NLP)
Improvements in NLP will allow AI systems to better understand and extract valuable information from unstructured data, such as financial reports, news articles, and social media posts. This will enhance the ability of financial forecasting models to capture and analyze qualitative information that could impact financial performance.
Predictive Analytics and Prescriptive Recommendations
AI-powered financial forecasting models will become more sophisticated, moving beyond simple predictions to providing prescriptive recommendations. These models will be able to suggest specific actions or strategies to optimize financial performance based on the forecasted scenarios.
Increased Automation and Real-Time Forecasting
As AI systems become more integrated into financial workflows, we can expect to see a greater degree of automation in the financial forecasting process. This will enable real-time forecasting and dynamic adjustments to account for changing market conditions and new information.
Explainable AI and Trustworthiness
There will be a growing emphasis on the development of “explainable AI” in financial forecasting, where the decision-making process of AI models can be more easily understood and trusted by human users. This will be crucial for compliance, regulatory, and ethical considerations.
Ethical and Responsible AI Practices
As the use of AI in financial forecasting becomes more widespread, there will be an increased focus on ensuring that these systems are developed and deployed in a responsible and ethical manner. This will include addressing issues such as bias, fairness, and privacy protection.
Conclusion
The integration of AI into financial forecasting has the potential to transform the way organizations plan, manage, and optimize their financial performance. By leveraging the power of AI, companies can achieve greater accuracy, speed, and personalization in their financial forecasts, ultimately leading to more informed decision-making and improved financial outcomes.
However, the successful implementation of AI in financial forecasting is not without its challenges. Organizations must address issues related to data quality, transparency, regulatory compliance, and talent development to fully harness the benefits of this transformative technology.
As AI continues to evolve and become more deeply integrated into financial workflows, we can expect to see even more exciting developments in the field of AI-driven financial forecasting. By staying informed and embracing these advancements, businesses can position themselves for success in an increasingly dynamic and competitive market.